Take a step again and have a look at your AI infrastructure. Can you say confidently that you’re arrange for AI success? And if you hear about generative AI, is your group and your infrastructure able to climate the winds of change.
In our on-demand webinar, Building Effective AI Infrastructure, three of our technical specialists lead a dialogue to reply your most urgent questions on your infrastructure. What makes an AI infrastructure profitable? What widespread errors do organizations make when constructing their infrastructure? What metrics must you use to measure success?
AI Infrastructure Means Including All the Things
AI infrastructure isn’t just about one resolution, and you’ll’t merely arrange a community and be finished with it. Rather, it ought to embody all of the methods and processes that cowl all the end-to-end lifecycle of AI tasks. This means being able to experiment with new use instances, put together datasets and options, and practice fashions and deploy them into manufacturing, in addition to monitoring the efficiency and accuracy of fashions. With these shifting components in place, you’ll lay the inspiration for achievement.
How Do You Build Effective Infrastructure?
Building efficient infrastructure is a balancing act consisting of three major parts: speedy experimentation, dependable productionization, and flexibility in an evolving ecosystem.
Experimentation
When it involves speedy experimentation of fashions, time is the important thing aspect. You need to have the ability to transfer shortly, and also you need your progress to be natural. You additionally wish to make knowledge entry simple for the important thing individuals in your workforce. Once you perceive the enterprise impression you’re on the lookout for, you’ll be able to work out your knowledge entry coverage.
To keep away from slowing down manufacturing and making expensive errors, it’s crucial to separate experimentation from manufacturing. This lets you iterate a lot quicker with out interrupting manufacturing operations. You must also ask a number of central questions: Is this a sound use case? Has each step been documented? Is it prepared for manufacturing?
Keep in thoughts that some instruments are higher than others and might save money and time. Look for repeatability in experimentation to make sure the integrity of your mannequin growth course of.
Production
Machine studying in manufacturing will make the belief that the info getting used for inference is just like the info it was educated on. You ought to anticipate that this assumption might be violated, both due to a change within the knowledge, exterior situations, or as a result of upstream software program methods have modified. You can shield your manufacturing pipeline with monitoring capabilities corresponding to knowledge drift, mannequin drift, and accuracy.
Collaboration throughout your group can be important to realizing worth at manufacturing scale, so you need to spend money on instruments and applied sciences that assist facilitate that cross-functional collaboration. Rather than knowledge scientists simply throwing a bunch of code over the fence to ML engineers, make sure that everybody understands the enterprise objective you’re attempting to attain. Then when issues change—as they inevitably do—you’ll be able to depend on this collaboration to hold your AI mission ahead and transfer your use case into manufacturing rather more shortly.
Adaptability
Things change. The world modifications, knowledge goes outdated shortly, and fashions begin to drift. When this occurs, you’ll must adapt shortly. One means to try this is to not anticipate perfection throughout the experimentation stage. Too many groups wait till they get a mannequin to perfection earlier than placing it into manufacturing, however this course of can lock them up for a yr or longer. If it’s taking you a yr to get your fashions to manufacturing, that’s too lengthy. If you deal with getting “good enough” fashions in lower than three months, you’ll be a way more nimble operation.
Focus on the use case. Think by way of the ROI you wish to obtain, which is able to aid you decide the place to make extra focused investments. Also, by specializing in small use instances and iterating on them shortly, you’ll be able to construct your infrastructure in order that your experimentation-to-production course of is repeatable.
Every time you introduce a brand new know-how, you need to do a autopsy and ask, what slowed us down? This will aid you assess your infrastructure and unlock better efficiencies.
Want to Learn More?
Listen to our on-demand webinar to search out out extra suggestions and tips from our knowledge science specialists about constructing the best AI infrastructure.
About the creator
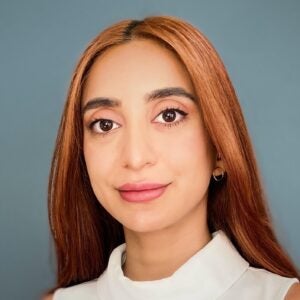
May Masoud is a knowledge scientist, AI advocate, and thought chief educated in classical Statistics and fashionable Machine Learning. At DataRobotic she designs market technique for the DataRobotic AI Platform, serving to world organizations derive measurable return on AI investments whereas sustaining enterprise governance and ethics.
May developed her technical basis by way of levels in Statistics and Economics, adopted by a Master of Business Analytics from the Schulich School of Business. This cocktail of technical and enterprise experience has formed May as an AI practitioner and a thought chief. May delivers Ethical AI and Democratizing AI keynotes and workshops for enterprise and tutorial communities.