In a latest research revealed in Nature Methods, researchers assessed a novel methodology for bacterial cell segmentation named Omnipose.
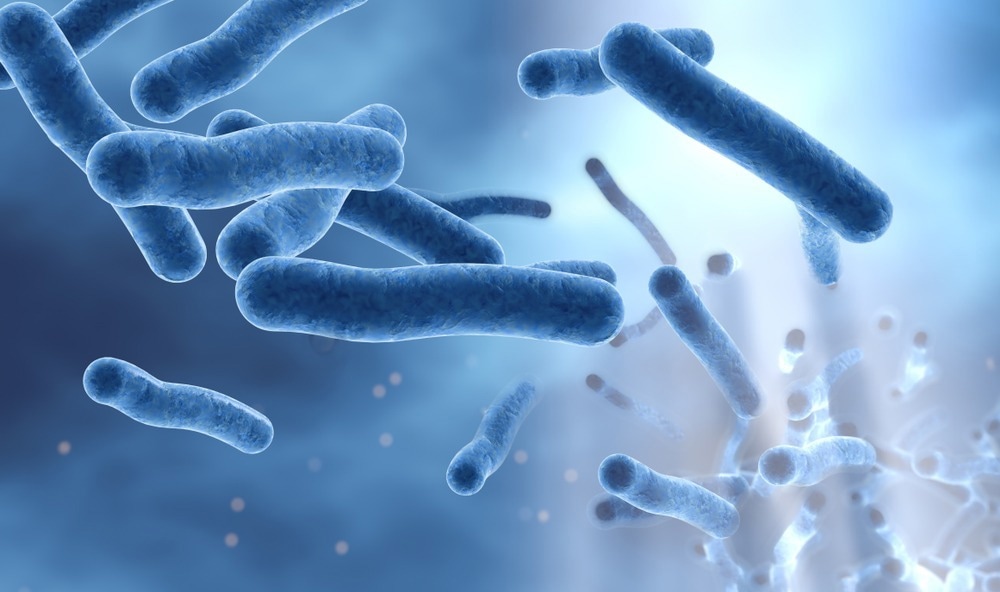
Background
Breakthroughs in microscopy are extraordinarily promising for enabling quantitative and correct measurements of morphological in addition to molecular phenomena in micro organism on the single-cell stage. However, this method is restrained by the accessibility and availability of strategies that may section cells with out relying on their optical or morphological properties.
Assessment of bacterial cell segmentation algorithms
In the current research, researchers assessed how state-of-the-art cell segmentation algorithms carried out on a wide range of bacterial cells.
The effectiveness of a number of picture segmentation methods which have been developed on bacterial cells has been documented. These strategies may be broadly divided into three teams:
- Conventional image-processing strategies
- Conventional/machine-learning hybrid strategies
- Deep neural networks (DNNs) strategies
The group sought to find efficient algorithms for additional analysis to create software program that may acknowledge micro organism globally. Due to the dearth of an intensive comparability between cell segmentation algorithms on bacterial cells, the group selected a number of examples from every group for this research: Mask R-CNN, StarDist, MiSiC, and Cellpose; Morphometrics; and SuperSegger.
Micrographs of many bacterial species exhibiting numerous morphologies and optical properties had been obtained for coaching and benchmarking these algorithms. Mutations or procedures that lead to excessive morphologies are regularly utilized in investigations of micro organism. Combinations of bacterial species that exhibit distinctive morphologies and visible properties had been photographed. The group gathered a complete of 4,833 photos or over 700,900 particular person cells from 14 completely different species. A fast methodology for manually annotating cells was developed and used on these images. The outcome was 47,000 representatively annotated cells, which had been used to create the ground-truth dataset (bact section).
Creation of a high-precision U-net segmentation algorithm
The group aimed to create a segmentation methodology that does not depend upon cell heart identification. The Cellpose framework, which may be damaged down into 5 primary components, resembling file processing, neural community structure, coaching objective features, community predictions, and masks reconstruction, served as the inspiration for the algorithm created within the current research. While enhancements had been made to every of those components, the masks reconstruction and community predictions are the 2 most vital breakthroughs within the developed system named Omnipose.
The distance area, also called the space rework, represents the separation between every level x inside a confined area and the purpose closest to the boundary. The cell chance output related to Cellpose is changed by the space area in Omnipose. Compared to different options that produce related fields, this leads to sooner convergence and improved numerical stability.
Omnipose demonstrated segmentation accuracy as a multifunctional segmentation software
The researchers educated a mannequin (bact section omni) on the bact section dataset in an effort to evaluate Omnipose’s efficiency towards it. Surprisingly, utilizing the comparative mannequin (bact section cp), Omnipose’s accuracy considerably outperforms that of Cellpose. This efficiency disparity between the algorithms is very apparent within the excessive IoU vary of 0.8-1.0. Bacteria are generally imaged at a calibrated pixel dimension of about 0.1 m, producing cells and cell labels which might be 5 to 50 pixels throughout. Bacteria are sometimes measured at scales between 0.5 and 5 m. IoU values above 0.8 are important for pixel-level accuracy in quantitative experiments at this scale. As a outcome, Omnipose is completely suited to finding out bacterial cells microscopically.
When educated on a consultant dataset, Omnipose may additionally section photographs acquired utilizing numerous modalities and comprising numerous topics. A dataset of 33,200 bacterial cells’ cytosol and membrane fluorescence was developed in an effort to take a look at Omnipose on photographs captured utilizing a modality apart from section distinction. The group found that Omnipose (bact fluor omni) outperformed Cellpose (bact fluor cp) when it comes to efficiency on morphologically assorted cells.
Next, the group explored how Omnipose could be used to section topics apart from micro organism. The generalist mannequin, which was educated on the bact section dataset and the worm dataset (worm bact omni) carried out in addition to or higher than the worm mannequin (worm omni). The group additionally used a personalized set of high-resolution C. elegans photographs to coach an Omnipose mannequin and found that the algorithm might successfully section these photographs regardless of their intricate underlying construction.
Conclusion
Overall, the research findings demonstrated that on combined bacterial cultures, cells handled with antibiotics, and cells with an elongated or branched form, Omnipose achieves commendable segmentation efficiency. Additionally, Omnipose’s benefits apply to non-bacterial topics, a variety of imaging modalities, and three-dimensional objects.