The use of synthetic intelligence (AI) within the funding sector is proving to be a major disruptor, catalyzing the connection between the completely different gamers and delivering a extra vivid image of the long run threat and alternatives throughout all completely different market segments. Real property investments usually are not an exception. In this text, we’ll showcase the flexibility of AI to enhance the standard of the potential funding’s future efficiency, with a particular instance from the true property phase.
The lack of transparency, effectivity, and sustainability in actual property at present is extra a rule than an exception. One may think that the rise in accessible information would result in larger transparency and extra environment friendly markets, however the reverse appears to be the case as elevated entry to huge quantities of information has made assessing actual property belongings rather more complicated.
In this context, an augmented intelligence strategy across the information can be more and more extra essential for asset managers, traders, and actual property builders to make sure a greater understanding of the true property belongings and take higher choices aimed toward optimizing each the Net Asset Value and the Net Operating Income. Yet, within the digital transformation period, the pricing and evaluation of actual property belongings is harder than described by brokers’ shows, valuation reviews, and conventional analytical approaches like hedonic fashions.
Previously, we demonstrated how DataRobot AI Platform permits traders, asset managers, and actual property builders to efficiently overcome a lot of the present challenges relating to the true property funding enterprise.
In this text, we’ll first take a better take a look at the idea of Real Estate Data Intelligence and the potential of AI to change into a recreation changer on this area of interest. We’ll then empirically take a look at this assumption primarily based on an instance of actual property asset evaluation. For this goal, we are going to showcase an end-to-end, data-driven strategy to cost predictions of actual property belongings via the DataRobot AI Platform.
Real Estate Data Intelligence
Today, essentially the most essential ‘raw material’ driving the true property market is information. Many actual property gamers have lengthy made choices primarily based on conventional information to reply the query of the standard of asset’s evaluation and an funding’s location inside a metropolis. This often concerned gathering market and property data, socio-economic information a few metropolis on a zipper code degree and data relating to entry to facilities (e.g., parks and eating places), and transportation networks. The conventional evaluation strategy additionally thought of components comparable to market instinct and expertise.
Although the quantity of information has been rising exponentially—internet hosting new variables which will make it potential to have a greater image of location’s future dangers and alternatives—the intelligence wanted to course of all this information and use it to learn actual property choices continues to be comparatively nascent.
Let’s assume that traders, asset managers, and actual property builders wish to consider an asset’s efficiency. While the influence of proximity is perhaps intuitive, residence costs and rents usually are not simply pushed by having close by facilities like top-tier eating places and academic amenities. Instead, they’re pushed by the entry to the suitable amount, combine and high quality of neighborhood options. More isn’t at all times higher. Nonlinear relationships between costs and facilities appear to be the rule somewhat than the exception throughout cities worldwide.
Also, the intersection of proximity and density to facilities varies amongst neighborhoods and cities. This candy spot has been obscured by a rising mass of latest accessible multimodal information (geospatial, time collection, textual content, and picture information) that’s more and more tough to tame, comparable to constructing vitality consumption spatially associated to different belongings in the identical zip code, variety of permits within the final 3 months issued to construct swimming swimming pools, Google evaluations for close by companies, and asset’s exterior photos captured by Google.
What would occur if an automatic intelligence machine strategy might course of and perceive all this more and more huge multimodal information via the lens of an actual property participant and use it to acquire fast actionable insights?
For instance, simply to call the enterprise of asset managers is mostly depending on these (however not restricted to) 4 fundamentals:
- Accurately estimating the present asset’s value and lease
- Estimating the expansion potential of a metropolis and neighborhood
- Automating and optimizing their funding technique
- Selling asset portfolios at a value that maximizes returns whereas minimizing time to market
However, they’re additionally concurrently coping with a number of challenges which will block them to acquire worthwhile and actionable enterprise insights. As mentioned within the earlier article, these challenges could embody:
- Automating the information preprocessing workflow of complicated and fragmented information
- Monitoring fashions in manufacturing and repeatedly studying in an automatic method, so being ready for actual property market shifts or sudden occasions.
Yet, when assessing property’s worth and the standard of funding’s location different key particular challenges come up, together with:
- Handling multimodal information comparable to photos, geospatial and textual content
- Building analytical approaches to evaluate asset’s value and lease that adjust to rules
- Treating prospects pretty and avoiding bias within the analytical strategy to estimate property’s worth.
From this viewpoint, one could maintain that if an automatic intelligence machine strategy can efficiently deal with all these challenges whereas matching the true property gamers’ enterprise expectations, this could change into an actual recreation changer for the trade as it’ll deliver intensive mild on the dialogue about actual property information intelligence: effectivity, transparency, location information, and actionable insights.
Predicting the Real Estate Asset’s Price Using DataRobotic
Processing Multimodal Datasets
Datarobot permits customers to simply mix a number of datasets right into a single coaching dataset for AI modeling. DataRobotic additionally processes almost each kind of information, comparable to satellite tv for pc and road imagery of actual property properties utilizing DataRobotic Visual AI, the latitude and longitude of properties and close by metropolis’s factors of curiosity utilizing DataRobotic Location AI, tweets, and evaluations with geotagged places utilizing DataRobotic Text AI. Recent historic traits in neighborhoods will also be seen with DataRobotic Feature Discovery and quite a lot of different particulars comparable to photo voltaic orientation, development 12 months, and vitality efficiency.
DataRobotic combines these datasets and information sorts into one coaching dataset used to construct machine studying fashions. In this educated instance, the purpose is to predict residence costs on the property degree within the metropolis of Madrid and the coaching dataset accommodates 5 completely different information sorts (numerical, categorical, textual content, location, and pictures) and +90 variables which are associated to those 5 completely different teams:
- Market efficiency
- Property efficiency
- Property options
- Neighborhood attributes
- City’s pulse (high quality and density of the factors of curiosity)
The wonderful thing about DataRobotic Explainable AI is that it spans all the platform. You can perceive the information and mannequin’s habits at any time. Once you utilize a coaching dataset, and after the Exploratory Data Analysis, DataRobotic flags any information high quality points and, if important points are spotlighted, will robotically deal with them within the modeling stage.
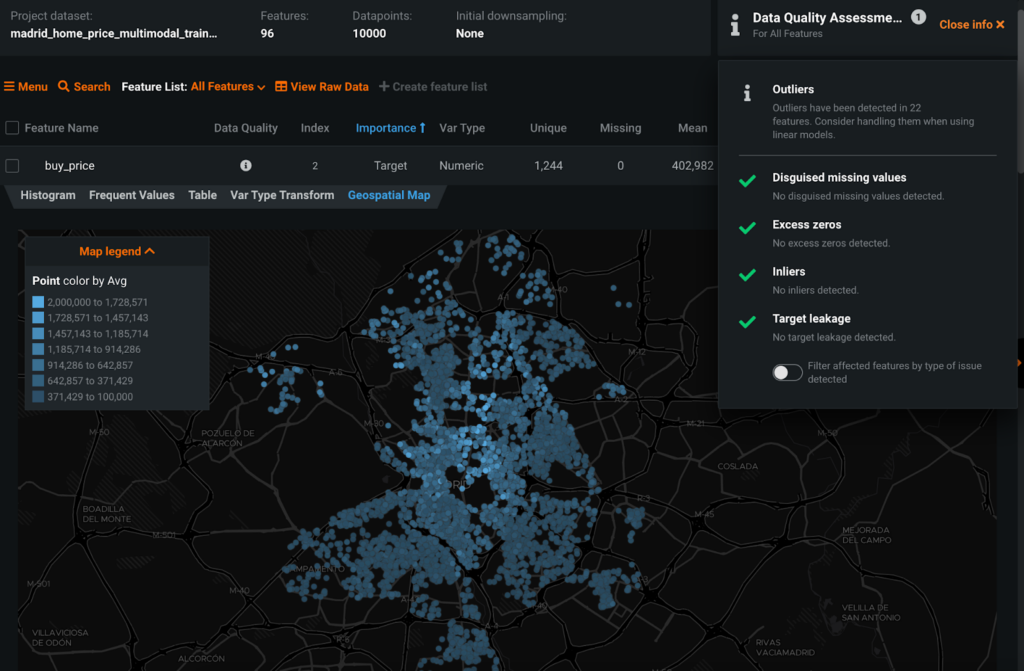
Rapid Modeling with DataRobotic AutoML
DataRobotic AutoML quickly builds and benchmarks lots of of modeling approaches utilizing personalized mannequin blueprints. Using built-in automation workflows, both via the no-code Graphical User Interface (GUI) or the code-centric DataRobot for information scientists, each information scientists and non-data scientists—comparable to asset managers and funding analysts—can construct, consider, perceive, clarify, and deploy their very own fashions.
Enabling picture augmentation generated the perfect outcomes for predicting home costs throughout the town of Madrid. DataRobotic robotically determines the perfect configuration for the dataset. However, we are able to customise it additional. As the determine beneath reveals, you may customise the picture augmentation flips, rotating, and scaling photos to extend the variety of observations for every object within the coaching dataset aimed to create excessive performing pc imaginative and prescient fashions.
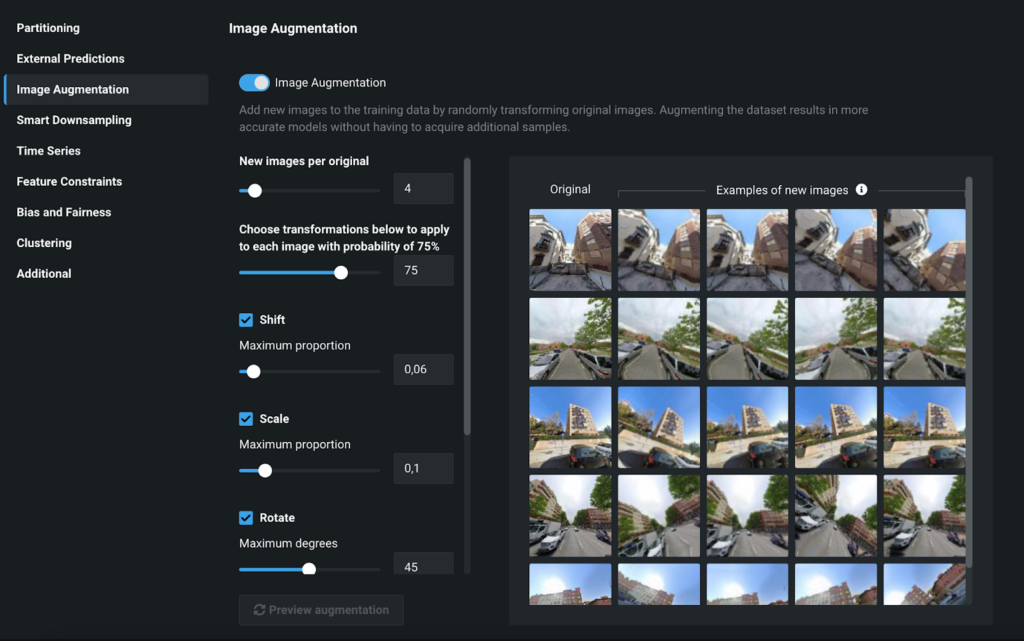
DataRobotic begins modeling after we allow some further settings, like together with superior ensembling and blueprints, in addition to seek for interactions to leverage relationships throughout a number of variables, probably yielding a greater mannequin accuracy and function constraints to combine the true property market experience and information.
In lower than an hour, DataRobotic produced a house-price multimodal mannequin that accurately predicted home costs throughout area and carried out particularly effectively at predicting which 10% of properties had the best residence costs. By utilizing this mannequin, all accuracy metrics would additionally adjust to nationwide valuation rules—as outlined by the Bank of Spain. For instance, the mannequin produced a RMSLE (Root Mean Squared Logarithmic Error) Cross Validation of 0.0825 and a MAPE (Mean Absolute Percentage Error) Cross Validation of 6.215. This would entail a roughly +/-€24,520 value distinction on common, in comparison with the true value, utilizing MAE (Mean Absolute Error) Cross Validation.
Understand & Explain Models with DataRobotic Trusted AI
DataRobotic AI Platform tries to bridge the hole between mannequin growth and enterprise choices whereas maximizing transparency at each step of the ML & AI lifecycle. As mentioned earlier, that is extremely essential for all actual property gamers, together with asset managers, as they should construct analytics approaches to evaluate asset sale and lease costs with none black-box patterns within the decision-making, delivering transparency in how predictions are generated.
So, let’s look beneath the hood at a few of DataRobotic Explainable AI performance that may be extra related for actual property gamers, permitting them to know the habits of fashions, encourage confidence of their outcomes, and simply translate these modeling outcomes into actionable enterprise insights and nice outcomes.
Accuracy over Space
Location AI and particularly, with the Accuracy Over Space explainability software, we are able to higher perceive how the house-price multimodal mannequin, developed in DataRobotic, is behaving on the native degree. Model accuracy can range significantly throughout geographic places—however, due to this explainability software—asset managers and funding analysts can shortly and precisely determine the place, by way of location, the mannequin is correct and the place it isn’t.
In the determine beneath, we see spatial match of our machine studying mannequin with most places the place the common residual is low and only a few places the place the mannequin is both over-predicting (see mild blue bars) or under-predicting (see mild pink bars), e.g., properties positioned close to Pozuelo de Alarcón.
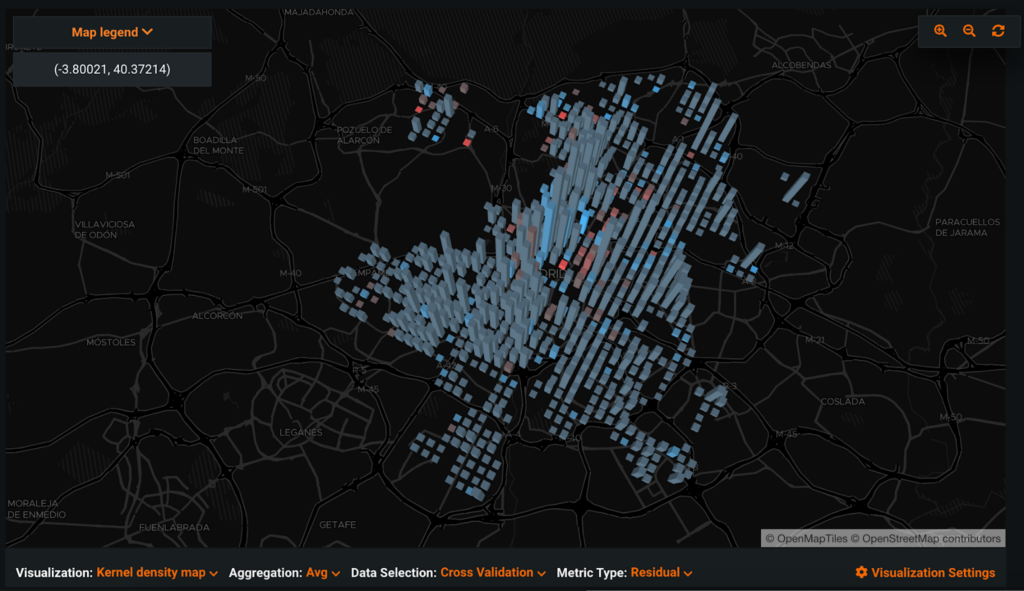
Global Explainability
One of the primary issues that actual property gamers often wish to perceive higher is the habits of the mannequin as an entire throughout all information. This is the place the interpretability capabilities of DataRobotic, like Feature Impact, Feature Effects, and Activation Maps—amongst others—come into play.
Feature Impact reveals crucial options of the mannequin’s predictions. DataRobotic can use both Permutation Based Importance or SHAP Importance to compute significance. It is value mentioning right here that when spatial construction is current within the coaching dataset, DataRobotic Location AI expands the normal automated function engineering to completely accommodate new geospatial variables for modeling that improves mannequin efficiency.
In the subsequent determine we see that among the many top-25 most essential options in essentially the most correct house-price multimodal mannequin, the metropolis’s facilities and location-based variables are essentially the most consultant. For instance, there’s a important influence from the common value (GEO_KNN_K10_LAG1_buy_price) and the kernel density common value (GEO_KNL_K10_LAG1_buy_price) of the primary ten nearest neighbors, in addition to facilities variables like proximity to each instructional and well being amenities.
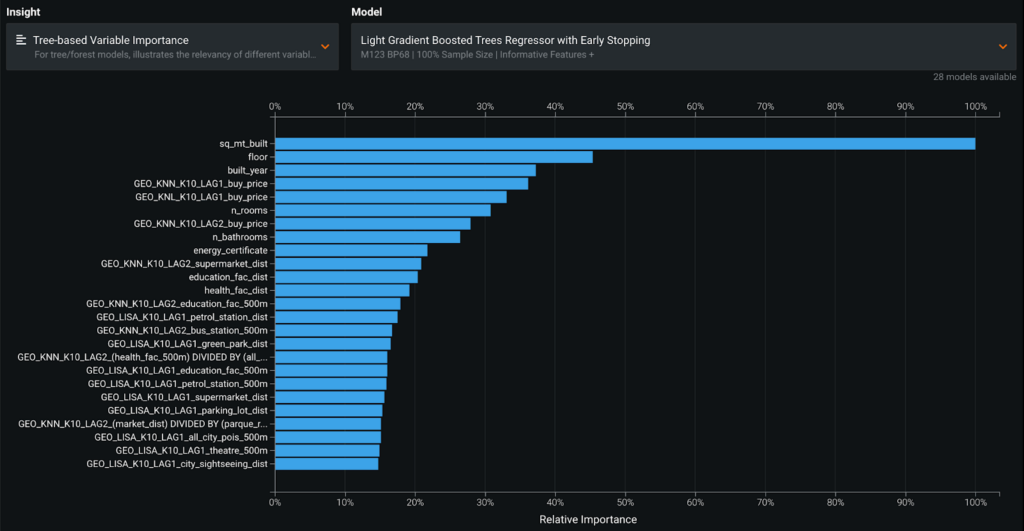
Once we all know which options are most influential to the mannequin’s choice making, actual property gamers will also be enthusiastic about addressing the query of how precisely do the options have an effect on the mannequin. This is precisely what you may deal with by utilizing Feature Effects, which permits DataRobotic customers to see how completely different values of a variable impacts the mannequin’s predictions. The calculation is predicated on Partial Dependence.
Looking on the Feature Effects of our prime mannequin, we are able to see, for instance, that larger vitality efficiency and being positioned nearer to Santiago Bernabéu Stadium (Real Madrid CF Stadium) result in greater common predicted home costs. These two insights match a fast gut-check: e.g., Santiago Bernabéu Stadium exerts a house value distance-decay impact over its neighboring areas as a result of it acts, coupled with Azca, as a serious sub-center of financial, retail, and leisure exercise in Madrid.
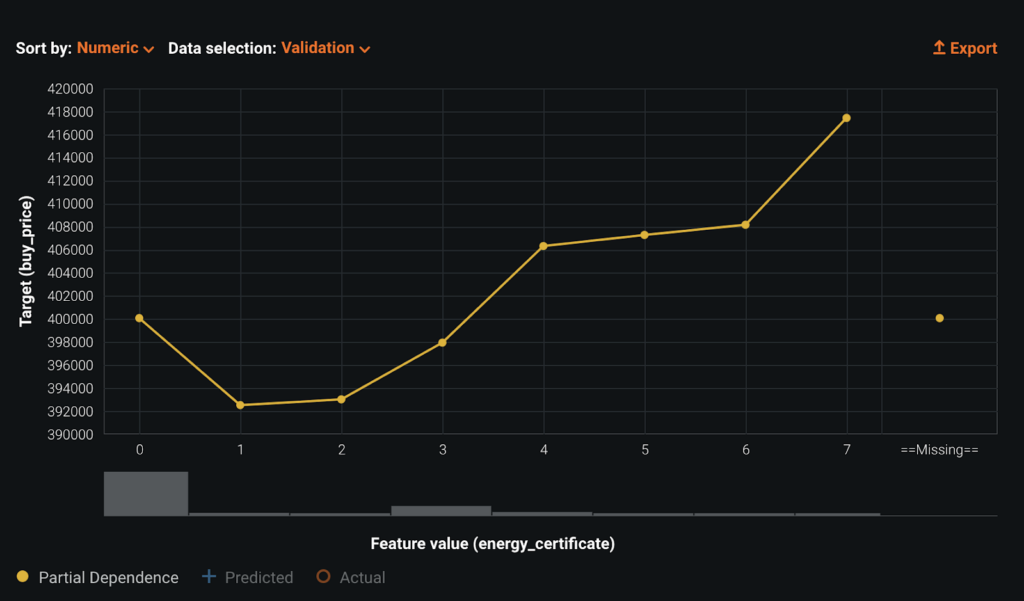
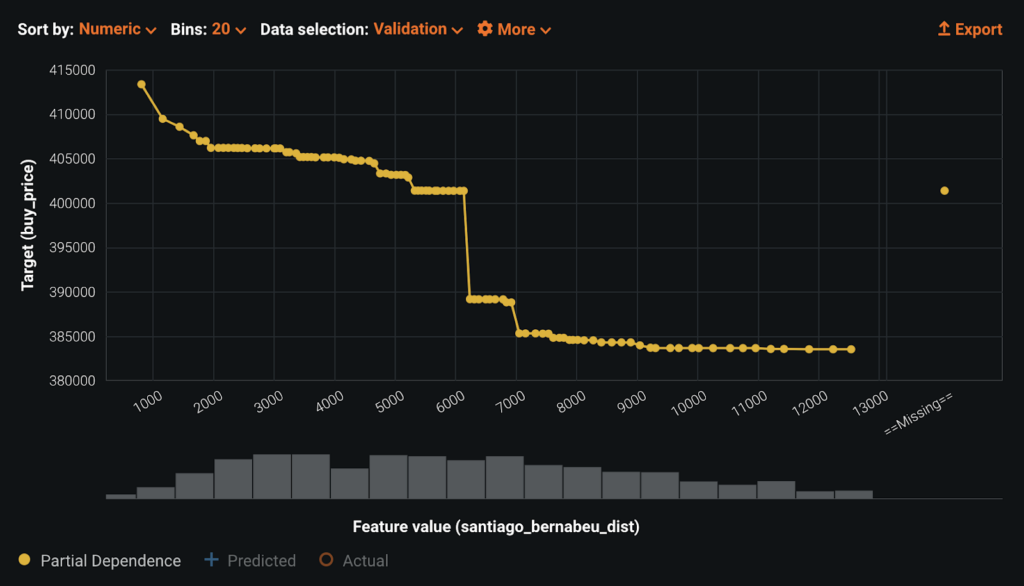
Because our coaching dataset is multimodal and accommodates imagery information of residential properties in Madrid, DataRobotic used machine studying fashions that comprise deep studying primarily based picture featurizers. Activation Maps permits DataRobotic customers to see which a part of numerous photos the machine studying mannequin is utilizing for making predictions. This may help actual property professionals decide if the machine studying mannequin is studying the appropriate data for the use case, doesn’t comprise undesired bias, and isn’t overfitting on spurious particulars.
Looking on the Activation Maps of our prime mannequin, we are able to observe that the mannequin is mostly targeted on the outside picture of properties. Of course, DataRobotic customers can simply customise the picture featurizer if essential.
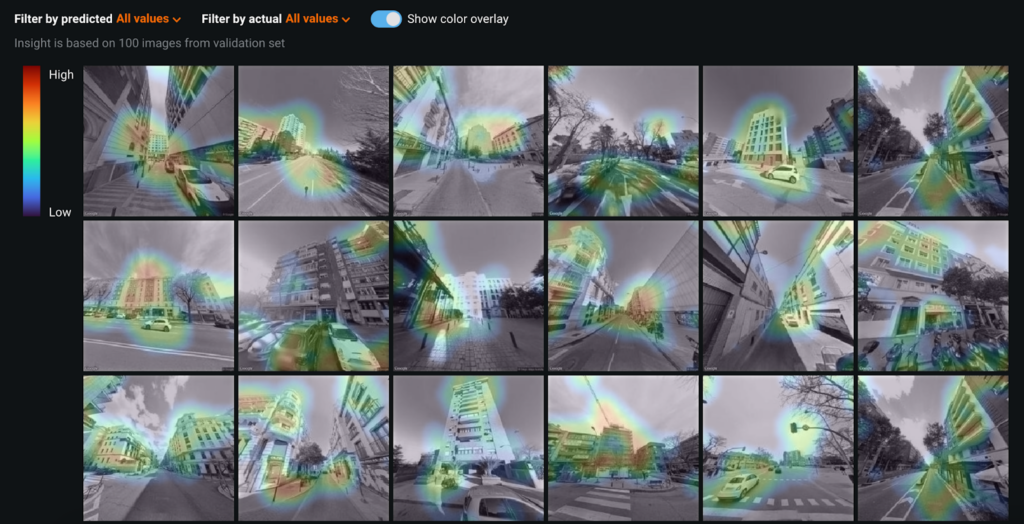
Local Explainability
After describing the general mannequin’s habits, actual property gamers and, particularly, asset managers and actual property appraisers, would most likely wish to know why a mannequin made a person prediction. This is extraordinarily worthwhile when it’s essential justify the choice an analytical mannequin has made. Also, when it’s essential optimize the true property product to develop in a particular location or the funding’s location choice inside a metropolis.
Let’s assume that, as an actual property developer, you wish to optimize the property’s value given a location in a metropolis whereas minimizing time on market. Local Explainability will assist them to determine the primary property’s worth contributors on the coaching time and subsequently operating each what-if eventualities and mathematical optimization on the scoring time by altering actionable options, e.g., residence dimension, variety of rooms and loos, and swimming pool development.
Local Explainability in DataRobot AI Platform is offered via Prediction Explanations. This will inform actual property professionals which options and values contributed to a person prediction—and their influence and the way a lot they contributed. DataRobotic can use both its personal XEMP explanations or SHAP explanations. Both forms of prediction explanations will be produced at coaching or scoring time.
Let’s have a better take a look at each prediction explanations sorts. In the primary determine beneath, utilizing our most correct house-price multimodal mannequin, we’re wanting on the XEMP prediction rationalization for row 7,621, which had a prediction of roughly €1,891,000 for residence gross sales value. The particular spatial location of this property, together with all associated geospatial variables (e.g., the common variety of instructional amenities inside 500 meters of the second ten nearest neighbors), and having 244 sq. meters, three loos, and 5 rooms had been the strongest contributors to this prediction. If we had been to make use of SHAP explanations (see second determine beneath) that might produce precise numbers for every function worth, which add as much as the entire predicted property’s sale value.
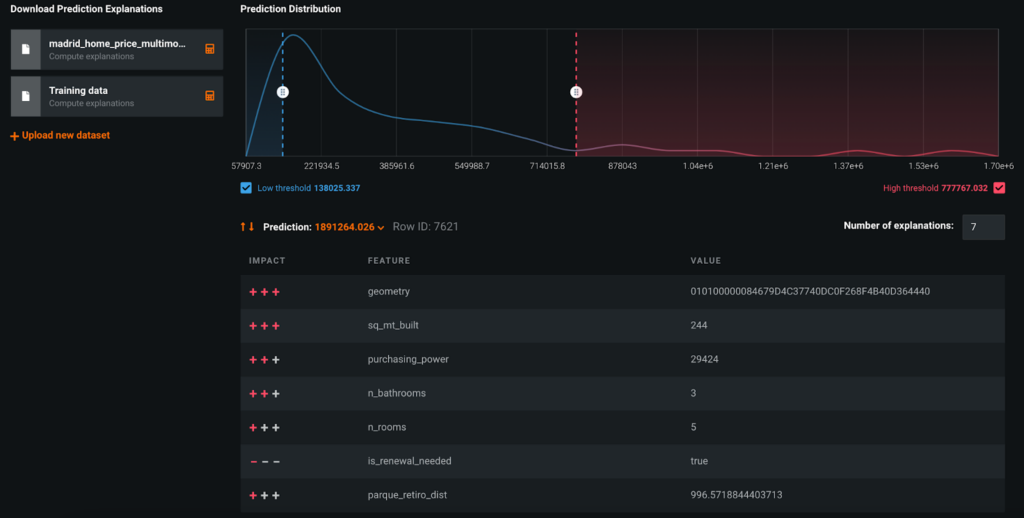
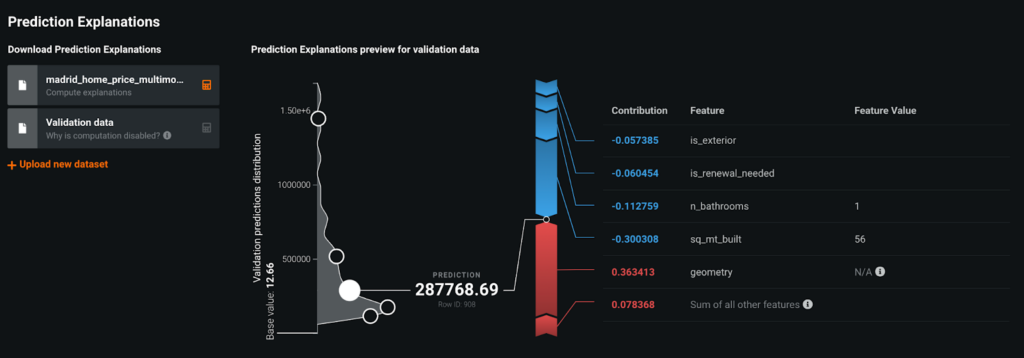
Compliant-Ready AI
With rules throughout numerous industries—and the true property sector not being an exception—the stress on actual property skilled groups to ship compliant-ready AI is bigger than ever. This often is the case, for instance, when asset managers or actual property servicers wish to assess the worth of Non-Performing Loans (NPL) portfolios or appraisers when finishing up property’s valuations that adjust to nationwide rules.
DataRobotic Automated Compliance Documentation permits to create automated customizable reviews primarily based on every step of the machine studying mannequin lifecycle with only a few clicks, thereby exponentially lowering the time-to-deployment whereas guaranteeing transparency and efficient mannequin threat administration.
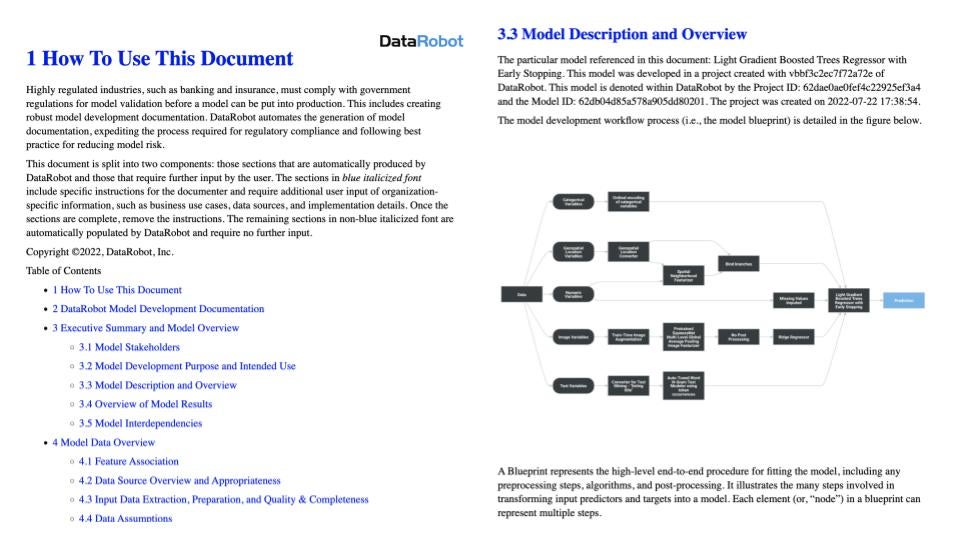
Consume Results with DataRobotic AI Applications
By bringing the advisable house-price multimodal mannequin to DataRobotic No Code AI Apps, actual property traders, asset managers, and builders can simply get clever AI Applications that automate the decision-making course of of their enterprise.
Within the AI App, actual property gamers can predict an actual property portfolio with 1000’s of belongings and dig deeper into the explanations driving every prediction with just a few clicks. They might additionally assess new places for both funding or actual property growth in addition to constructing their very own reporting dashboards. As their core enterprise is predicated on the standard of asset’s evaluation and an funding’s location, these AI Application’s examples could be particularly worthwhile for asset managers, actual property providers, valuation advisory corporations, and actual property builders.
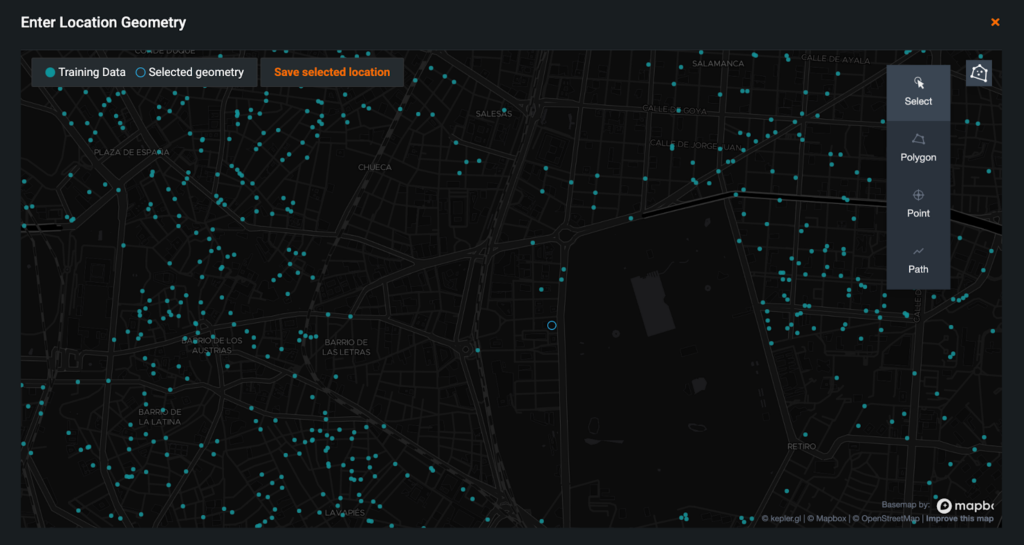
Interestingly, actual property gamers can even create their very own eventualities primarily based on their instinct and information of the market to benchmark mannequin outputs or construct optimization fashions that both maximize or reduce their enterprise outcomes. This additionally would assist them to automate their funding and growth technique.
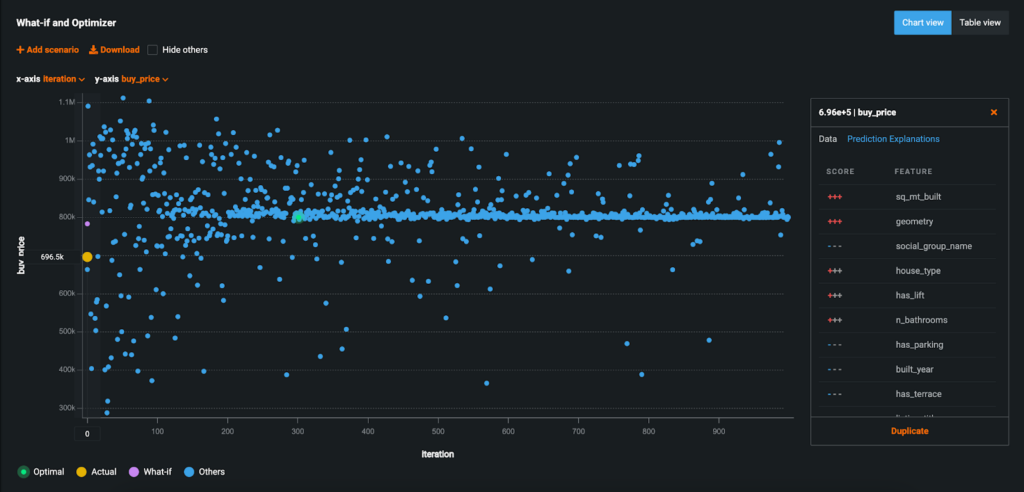
For instance, asset managers will be capable to promote asset portfolios at a value that maximizes returns whereas minimizing time to market. Likewise, actual property builders will be capable to add new property value eventualities in several metropolis places by altering these actionable variables of their curiosity (e.g., residence dimension, variety of rooms) or constructing optimization fashions to maximise particular outcomes given sure enterprise and market constraints (e.g., discovering the perfect actual property product configuration to go to market with, given sure market value circumstances). DataRobotic will quickly generate new insights aimed toward serving to actual property gamers to have full flexibility in testing completely different potential conditions, eventualities, and optimum enterprise outcomes as we are able to see beneath.
Last however not least, superior analytics groups might additionally benefit from the code-centric DataRobotic performance to construct their very own code-based purposes. An instance of code-based utility is proven beneath. With the usage of DataRobotic API, superior analytics groups in the true property sector will be capable to simply construct AI purposes in days that might do the next :
- Accurately predict the property’s value for a single asset or portfolio and a brand new location, whereas digging deeper into the explanations driving every prediction
- Estimate the long run actual property market modifications (e.g., costs and rents over the subsequent 12 months) and the expansion potential of neighborhoods, districts, and cities
- Search and benchmark potential funding’s places in opposition to actual property comparables
- Either maximize or reduce enterprise outcomes via optimization fashions
- Automate their enterprise technique and decision-making course of
Conclusions
We have simply proven how AI can foster and scale Augmented Intelligence in funding and actual property by exhibiting howDataRobotic shortly produced a scalable and clear end-to-end analytics strategy to cost predictions of actual property belongings, whereas guaranteeing transparency and efficient mannequin threat administration at each step of the ML & AI lifecycle.
DataRobot AI Platform is ready to analyze all kinds of patterns and make predictions primarily based on the information that’s being analyzed. This is essential, as the true property sector additionally has main enterprise challenges which will require the usage of different ML & AI approaches, like unsupervised studying (multimodal clustering and time collection anomaly detection) to efficiently deal with them. AI will also be utilized to quite a few different worthwhile use circumstances in the true property sector and past the residing actual property phase. Examples embody each the workplace and retail market segments, in addition to use circumstances associated to traders, property managers, and business tenants. For occasion, use circumstances associated to optimizing the leasing portfolio administration, like predicting which tenants will renew and which of them will go away the property when their lease expires—thereby serving to to take care of the next occupancy fee and foster a larger Net Operating Income (NOI).
About the creator
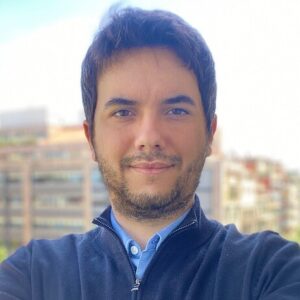
Senior Customer-Facing Data Scientist, Team Lead at DataRobotic
Jaume is a Senior Customer-Facing Data Scientist, Team Lead at DataRobotic. He leverages the AI Cloud DataRobotic platform to drive the adoption of AI and machine studying in main enterprises in EMEA, with a particular focus inside the Banking, Insurance, and Utilities Industries. Prior to DataRobotic, Jaume labored in quite a lot of data-centric roles in universities and main enterprises for greater than 10 years, each as a person contributor and director of information science. During this time interval, he had the chance to construct and lead many analytics merchandise and information advisory providers, with a particular give attention to the Real Estate sector, leveraging machine studying and utilized statistics.
Jaume holds a PhD. in Statistics and Operations Research from UPC-BarcelonaTech and a PhD in Urban and Regional Economics from TU Delft and in his spare time enjoys touring and enjoying chess.