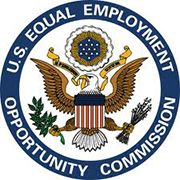
By AI Trends Staff
While AI in hiring is now extensively used for writing job descriptions, screening candidates, and automating interviews, it poses a threat of large discrimination if not carried out fastidiously.
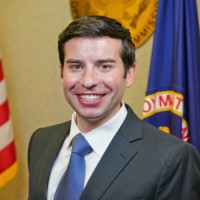
That was the message from Keith Sonderling, Commissioner with the US Equal Opportunity Commision, talking on the AI World Government occasion held stay and just about in Alexandria, Va., final week. Sonderling is accountable for implementing federal legal guidelines that prohibit discrimination towards job candidates due to race, coloration, faith, intercourse, nationwide origin, age or incapacity.
“The thought that AI would become mainstream in HR departments was closer to science fiction two year ago, but the pandemic has accelerated the rate at which AI is being used by employers,” he stated. “Virtual recruiting is now here to stay.”
It’s a busy time for HR professionals. “The great resignation is leading to the great rehiring, and AI will play a role in that like we have not seen before,” Sonderling stated.
AI has been employed for years in hiring—“It did not happen overnight.”—for duties together with chatting with purposes, predicting whether or not a candidate would take the job, projecting what sort of worker they might be and mapping out upskilling and reskilling alternatives. “In short, AI is now making all the decisions once made by HR personnel,” which he didn’t characterize nearly as good or unhealthy.
“Carefully designed and properly used, AI has the potential to make the workplace more fair,” Sonderling stated. “But carelessly implemented, AI could discriminate on a scale we have never seen before by an HR professional.”
Training Datasets for AI Models Used for Hiring Need to Reflect Diversity
This is as a result of AI fashions depend on coaching knowledge. If the corporate’s present workforce is used as the idea for coaching, “It will replicate the status quo. If it’s one gender or one race primarily, it will replicate that,” he stated. Conversely, AI will help mitigate dangers of hiring bias by race, ethnic background, or incapacity standing. “I want to see AI improve on workplace discrimination,” he stated.
Amazon started constructing a hiring utility in 2014, and located over time that it discriminated towards ladies in its suggestions, as a result of the AI mannequin was skilled on a dataset of the corporate’s personal hiring file for the earlier 10 years, which was primarily of males. Amazon builders tried to right it however in the end scrapped the system in 2017.
Facebook has not too long ago agreed to pay $14.25 million to settle civil claims by the US authorities that the social media firm discriminated towards American staff and violated federal recruitment guidelines, based on an account from Reuters. The case centered on Facebook’s use of what it referred to as its PERM program for labor certification. The authorities discovered that Facebook refused to rent American staff for jobs that had been reserved for short-term visa holders underneath the PERM program.
“Excluding people from the hiring pool is a violation,” Sonderling stated. If the AI program “withholds the existence of the job opportunity to that class, so they cannot exercise their rights, or if it downgrades a protected class, it is within our domain,” he stated.
Employment assessments, which turned extra frequent after World War II, have offered excessive worth to HR managers and with assist from AI they’ve the potential to attenuate bias in hiring. “At the same time, they are vulnerable to claims of discrimination, so employers need to be careful and cannot take a hands-off approach,” Sonderling stated. “Inaccurate data will amplify bias in decision-making. Employers must be vigilant against discriminatory outcomes.”
He really useful researching options from distributors who vet knowledge for dangers of bias on the idea of race, intercourse, and different elements.
One instance is from HireVue of South Jordan, Utah, which has constructed a hiring platform predicated on the US Equal Opportunity Commission’s Uniform Guidelines, designed particularly to mitigate unfair hiring practices, based on an account from allWork.
A submit on AI moral ideas on its web site states partially, “Because HireVue uses AI technology in our products, we actively work to prevent the introduction or propagation of bias against any group or individual. We will continue to carefully review the datasets we use in our work and ensure that they are as accurate and diverse as possible. We also continue to advance our abilities to monitor, detect, and mitigate bias. We strive to build teams from diverse backgrounds with diverse knowledge, experiences, and perspectives to best represent the people our systems serve.”
Also, “Our data scientists and IO psychologists build HireVue Assessment algorithms in a way that removes data from consideration by the algorithm that contributes to adverse impact without significantly impacting the assessment’s predictive accuracy. The result is a highly valid, bias-mitigated assessment that helps to enhance human decision making while actively promoting diversity and equal opportunity regardless of gender, ethnicity, age, or disability status.”
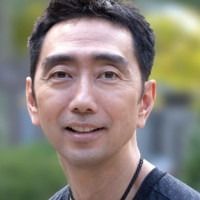
The subject of bias in datasets used to coach AI fashions will not be confined to hiring. Dr. Ed Ikeguchi, CEO of AiCure, an AI analytics firm working within the life sciences trade, acknowledged in a current account in HealthcareITNews, “AI is only as strong as the data it’s fed, and lately that data backbone’s credibility is being increasingly called into question. Today’s AI developers lack access to large, diverse data sets on which to train and validate new tools.”
He added, “They often need to leverage open-source datasets, but many of these were trained using computer programmer volunteers, which is a predominantly white population. Because algorithms are often trained on single-origin data samples with limited diversity, when applied in real-world scenarios to a broader population of different races, genders, ages, and more, tech that appeared highly accurate in research may prove unreliable.”
Also, “There needs to be an element of governance and peer review for all algorithms, as even the most solid and tested algorithm is bound to have unexpected results arise. An algorithm is never done learning—it must be constantly developed and fed more data to improve.”
And, “As an industry, we need to become more skeptical of AI’s conclusions and encourage transparency in the industry. Companies should readily answer basic questions, such as ‘How was the algorithm trained? On what basis did it draw this conclusion?”
Read the supply articles and data at AI World Government, from Reuters and from HealthcareITNews.