Listen to this text |
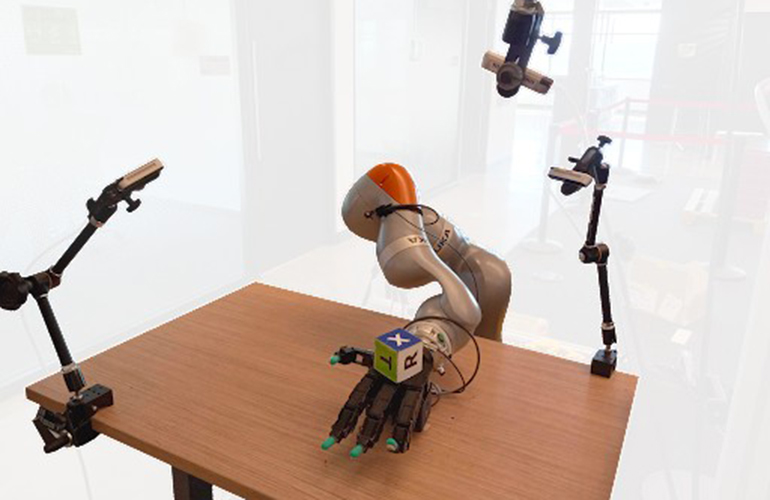
The setup for NVIDIA’s DeXtreme undertaking utilizing a Kuka robotic arm and an Allegro Hand. | Source: NVIDIA
Robotic arms are notoriously advanced and tough to regulate. The human arms they imitate include 27 completely different bones, 27 joints and 34 muscle mass, all working collectively to assist us carry out our day by day duties. Translating this course of into robotics is tougher than creating robots that use legs to stroll, for instance.
Methods usually used to show robotic management, like conventional strategies with exactly pre-programmed grasps and motions or deep reinforcement studying (RL) strategies, fall brief on the subject of working a robotic hand.
Pre-programmed motions are too restricted for the generalized duties a robotic hand would ideally be capable to carry out, and deep RL strategies that prepare neural networks to regulate robotic joints require thousands and thousands, or billions, of real-world samples to study from.
NVIDIA, as a substitute, used its Isaac Gym RL robotics simulator to coach an Allegro Hand, a light-weight, anthropomorphic robotic hand with three off-the-shelf cameras connected, as a part of its DeXtreme undertaking. The Isaac simulator is ready to run greater than simulations 10,000 occasions sooner than the true world, in line with the corporate, whereas nonetheless obeying the legal guidelines of physics.
With Isaac Gym, NVIDIA was in a position to train the Allegro Hand to control a dice and match supplied goal positions, orientations or poses. NVIDIA’s neural community mind discovered to do all of this in simulation after which the workforce transplanted it to regulate a robotic in the true world.
Training the neural community
In addition to its end-to-end simulation surroundings Isaac Gym, NVIDIA used its PhysX simulator, which simulates the world on the GPU that stays within the GPU reminiscence whereas the deep studying management coverage community is being educated, to coach the hand.
Training in simulations gives a number of advantages for robotics. Besides NVIDIA’s capability to run simulations a lot sooner than they’d play out in the true world, robotic {hardware} is liable to breaking after quite a lot of use.
According to NVIDIA, the workforce working with the hand typically needed to cease to restore the robotic hand, issues like tightening screws, changing ribbon cables and resting the hand to let it cool, after extended use. This makes it tough to get the type of coaching the robotic wants in the true world.
To prepare the robotic’s neural community, NVIDIA’s Omniverse Replicator generated round 5 million frames of artificial knowledge, which means NVIDIA’s workforce didn’t have to make use of any actual pictures. With NVIDIA’s coaching methodology, a neural community is educated utilizing a way referred to as area randomization, which adjustments lighting and digicam positions to present the community extra strong capabilities.
All of the coaching was executed on a single Omniverse OVX server, and the system can train a very good coverage in about 32 hours. According to NVIDIA, it might take a robotic 42 years to get the identical expertise in the true world.