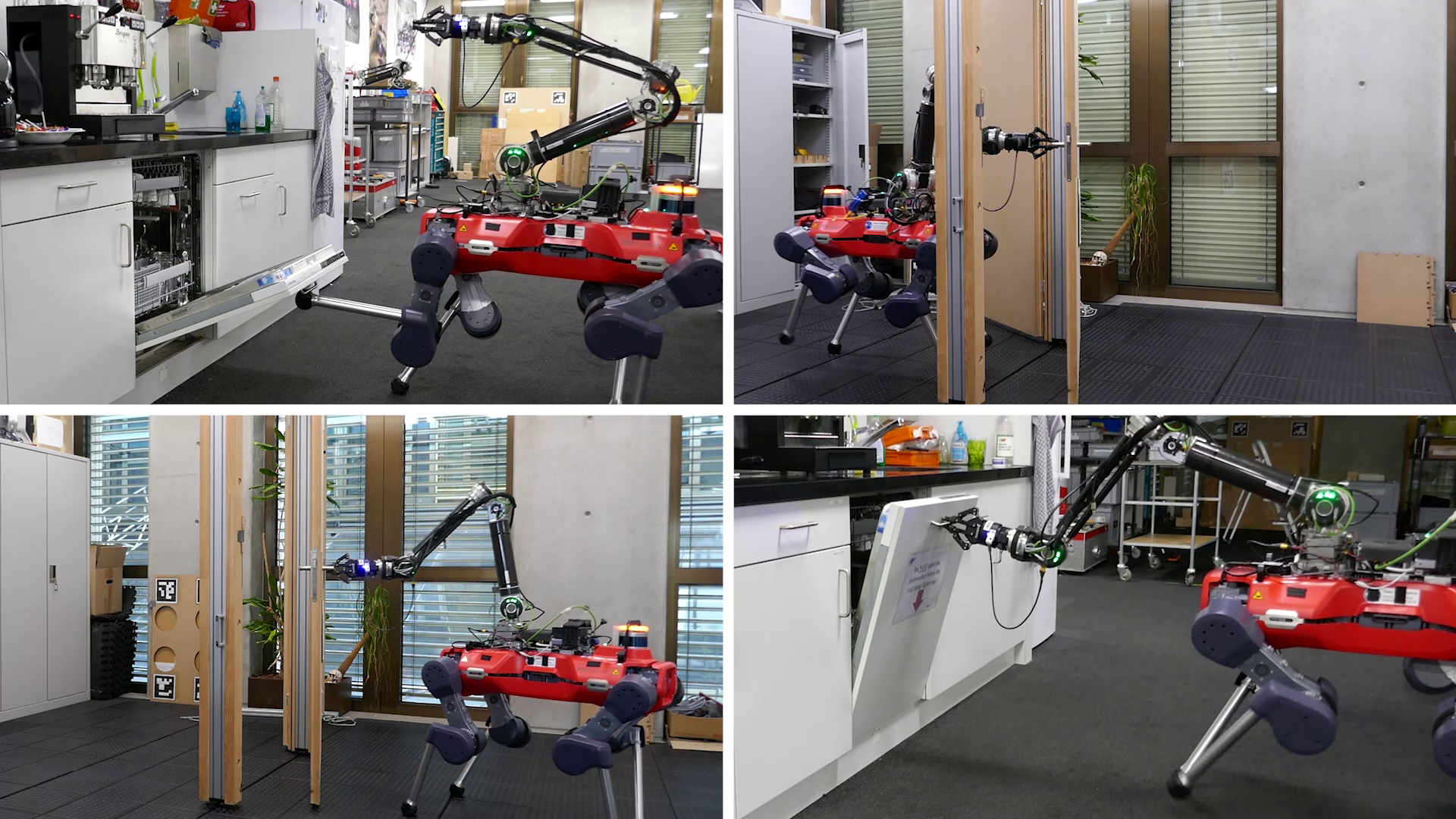
Picture from paper “Versatile multicontact planning and management for legged loco-manipulation“. © American Association for the Advancement of Science
We had the possibility to interview Jean Pierre Sleiman, creator of the paper “Versatile multicontact planning and control for legged loco-manipulation”, lately revealed in Science Robotics.
What is the subject of the analysis in your paper?
The analysis subject focuses on creating a model-based planning and management structure that allows legged cellular manipulators to sort out numerous loco-manipulation issues (i.e., manipulation issues inherently involving a locomotion component). Our examine particularly focused duties that will require a number of contact interactions to be solved, relatively than pick-and-place purposes. To guarantee our method is just not restricted to simulation environments, we utilized it to unravel real-world duties with a legged system consisting of the quadrupedal platform ANYmal geared up with DynaArm, a custom-built 6-DoF robotic arm.
Could you inform us concerning the implications of your analysis and why it’s an attention-grabbing space for examine?
The analysis was pushed by the need to make such robots, specifically legged cellular manipulators, able to fixing quite a lot of real-world duties, equivalent to traversing doorways, opening/closing dishwashers, manipulating valves in an industrial setting, and so forth. An ordinary method would have been to sort out every job individually and independently by dedicating a considerable quantity of engineering effort to handcraft the specified behaviors:
This is usually achieved via the usage of hard-coded state-machines during which the designer specifies a sequence of sub-goals (e.g., grasp the door deal with, open the door to a desired angle, maintain the door with one of many toes, transfer the arm to the opposite aspect of the door, cross via the door whereas closing it, and so on.). Alternatively, a human knowledgeable might exhibit tips on how to clear up the duty by teleoperating the robotic, recording its movement, and having the robotic be taught to imitate the recorded conduct.
However, this course of may be very gradual, tedious, and vulnerable to engineering design errors. To keep away from this burden for each new job, the analysis opted for a extra structured method within the type of a single planner that may mechanically uncover the required behaviors for a variety of loco-manipulation duties, with out requiring any detailed steering for any of them.
Could you clarify your methodology?
The key perception underlying our methodology was that all the loco-manipulation duties that we aimed to unravel might be modeled as Task and Motion Planning (TAMP) issues. TAMP is a well-established framework that has been primarily used to unravel sequential manipulation issues the place the robotic already possesses a set of primitive expertise (e.g., decide object, place object, transfer to object, throw object, and so on.), however nonetheless has to correctly combine them to unravel extra complicated long-horizon duties.
This perspective enabled us to plot a single bi-level optimization formulation that may embody all our duties, and exploit domain-specific data, relatively than task-specific data. By combining this with the well-established strengths of various planning methods (trajectory optimization, knowledgeable graph search, and sampling-based planning), we had been capable of obtain an efficient search technique that solves the optimization downside.
The fundamental technical novelty in our work lies within the Offline Multi-Contact Planning Module, depicted in Module B of Figure 1 within the paper. Its total setup might be summarized as follows: Starting from a user-defined set of robotic end-effectors (e.g., entrance left foot, entrance proper foot, gripper, and so on.) and object affordances (these describe the place the robotic can work together with the article), a discrete state that captures the mixture of all contact pairings is launched. Given a begin and purpose state (e.g., the robotic ought to find yourself behind the door), the multi-contact planner then solves a single-query downside by incrementally rising a tree through a bi-level search over possible contact modes collectively with steady robot-object trajectories. The ensuing plan is enhanced with a single long-horizon trajectory optimization over the found contact sequence.
What had been your fundamental findings?
We discovered that our planning framework was capable of quickly uncover complicated multi- contact plans for numerous loco-manipulation duties, regardless of having offered it with minimal steering. For instance, for the door-traversal situation, we specify the door affordances (i.e., the deal with, again floor, and entrance floor), and solely present a sparse goal by merely asking the robotic to finish up behind the door. Additionally, we discovered that the generated behaviors are bodily constant and might be reliably executed with an actual legged cellular manipulator.
What additional work are you planning on this space?
We see the introduced framework as a stepping stone towards creating a totally autonomous loco-manipulation pipeline. However, we see some limitations that we purpose to deal with in future work. These limitations are primarily related to the task-execution section, the place monitoring behaviors generated on the premise of pre-modeled environments is barely viable below the idea of a fairly correct description, which isn’t all the time simple to outline.
Robustness to modeling mismatches might be vastly improved by complementing our planner with data-driven methods, equivalent to deep reinforcement studying (DRL). So one attention-grabbing path for future work could be to information the coaching of a strong DRL coverage utilizing dependable knowledgeable demonstrations that may be quickly generated by our loco-manipulation planner to unravel a set of difficult duties with minimal reward-engineering.
About the creator
![]() |
Jean-Pierre Sleiman obtained the B.E. diploma in mechanical engineering from the American University of Beirut (AUB), Lebanon, in 2016, and the M.S. diploma in automation and management from Politecnico Di Milano, Italy, in 2018. He is at the moment a Ph.D. candidate on the Robotic Systems Lab (RSL), ETH Zurich, Switzerland. His present analysis pursuits embrace optimization-based planning and management for legged cellular manipulation. |
Daniel Carrillo-Zapata
was awared his PhD in swarm robotics on the Bristol Robotics Lab in 2020. He now fosters the tradition of “scientific agitation” to interact in two-way conversations between researchers and society.
Daniel Carrillo-Zapata
was awared his PhD in swarm robotics on the Bristol Robotics Lab in 2020. He now fosters the tradition of “scientific agitation” to interact in two-way conversations between researchers and society.