Listen to this text |
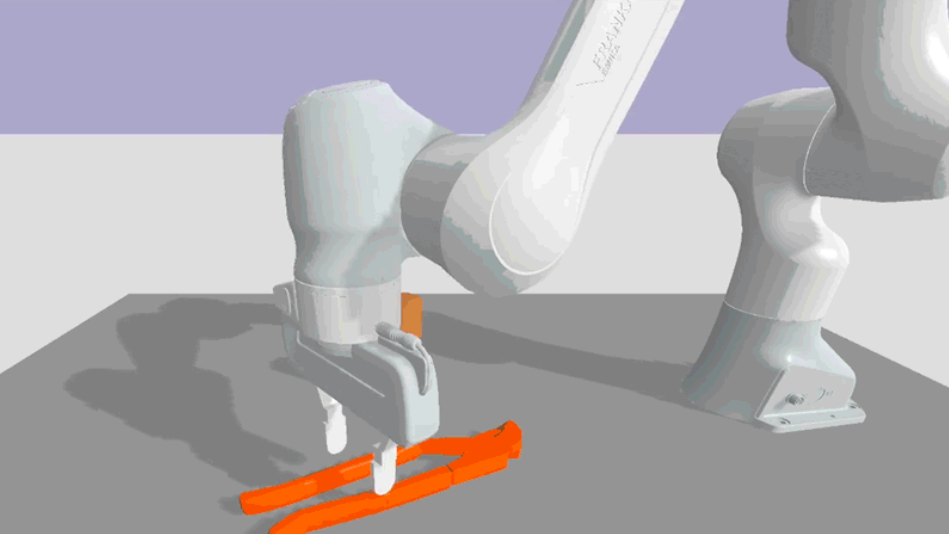
Researchers discovered human language descriptions of instruments accelerated the educational of simulated robotic arms. | Credit: Princeton University
Exploring a brand new option to educate robots, Princeton researchers have discovered that human-language descriptions of instruments can speed up the educational of a simulated robotic arm lifting and utilizing quite a lot of instruments.
The outcomes construct on proof that offering richer data throughout synthetic intelligence (AI) coaching could make autonomous robots extra adaptive to new conditions, bettering their security and effectiveness.
Adding descriptions of a software’s kind and performance to the coaching course of for the robotic improved the robotic’s potential to control newly encountered instruments that weren’t within the authentic coaching set. A staff of mechanical engineers and laptop scientists offered the brand new technique, Accelerated Learning of Tool Manipulation with LAnguage, or ATLA, on the Conference on Robot Learning.
Robotic arms have nice potential to assist with repetitive or difficult duties, however coaching robots to control instruments successfully is tough: Tools have all kinds of shapes, and a robotic’s dexterity and imaginative and prescient aren’t any match for a human’s.
“Extra information in the form of language can help a robot learn to use the tools more quickly,” mentioned research coauthor Anirudha Majumdar, an assistant professor of mechanical and aerospace engineering at Princeton who leads the Intelligent Robot Motion Lab.
The staff obtained software descriptions by querying GPT-3, a big language mannequin launched by OpenAI in 2020 that makes use of a type of AI known as deep studying to generate textual content in response to a immediate. After experimenting with varied prompts, they settled on utilizing “Describe the [feature] of [tool] in a detailed and scientific response,” the place the characteristic was the form or function of the software.
“Because these language models have been trained on the internet, in some sense you can think of this as a different way of retrieving that information,” extra effectively and comprehensively than utilizing crowdsourcing or scraping particular web sites for software descriptions, mentioned Karthik Narasimhan, an assistant professor of laptop science and coauthor of the research. Narasimhan is a lead school member in Princeton’s pure language processing (NLP) group, and contributed to the unique GPT language mannequin as a visiting analysis scientist at OpenAI.
This work is the primary collaboration between Narasimhan’s and Majumdar’s analysis teams. Majumdar focuses on growing AI-based insurance policies to assist robots – together with flying and strolling robots – generalize their capabilities to new settings, and he was curious in regards to the potential of latest “massive progress in natural language processing” to profit robotic studying, he mentioned.
For their simulated robotic studying experiments, the staff chosen a coaching set of 27 instruments, starting from an axe to a squeegee. They gave the robotic arm 4 totally different duties: push the software, raise the software, use it to brush a cylinder alongside a desk, or hammer a peg right into a gap. The researchers developed a collection of insurance policies utilizing machine studying coaching approaches with and with out language data, after which in contrast the insurance policies’ efficiency on a separate take a look at set of 9 instruments with paired descriptions.
This method is named meta-learning, because the robotic improves its potential to be taught with every successive process. It’s not solely studying to make use of every software, but in addition “trying to learn to understand the descriptions of each of these hundred different tools, so when it sees the 101st tool it’s faster in learning to use the new tool,” mentioned Narasimhan. “We’re doing two things: We’re teaching the robot how to use the tools, but we’re also teaching it English.”
The researchers measured the success of the robotic in pushing, lifting, sweeping and hammering with the 9 take a look at instruments, evaluating the outcomes achieved with the insurance policies that used language within the machine studying course of to those who didn’t use language data. In most instances, the language data supplied vital benefits for the robotic’s potential to make use of new instruments.
One process that confirmed notable variations between the insurance policies was utilizing a crowbar to brush a cylinder, or bottle, alongside a desk, mentioned Allen Z. Ren, a Ph.D. scholar in Majumdar’s group and lead writer of the analysis paper.
“With the language training, it learns to grasp at the long end of the crowbar and use the curved surface to better constrain the movement of the bottle,” mentioned Ren. “Without the language, it grasped the crowbar close to the curved surface and it was harder to control.”
The analysis was supported partially by the Toyota Research Institute (TRI), and is an element of a bigger TRI-funded undertaking in Majumdar’s analysis group geared toward bettering robots’ potential to operate in novel conditions that differ from their coaching environments.
“The broad goal is to get robotic systems – specifically, ones that are trained using machine learning — to generalize to new environments,” mentioned Majumdar. Other TRI-supported work by his group has addressed failure prediction for vision-based robotic management, and used an “adversarial environment generation” method to assist robotic insurance policies operate higher in circumstances outdoors their preliminary coaching.
Editor’s Note: This article was republished from Princeton University.