Listen to this text |
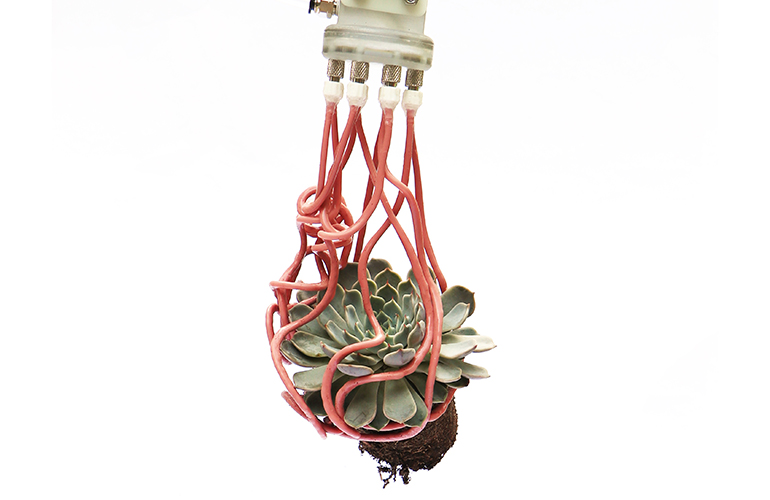
Harvard’s tentacle-like gripper wrapping round a succulent. | Source: Harvard Microrobotics Lab/Harvard SEAS
Researchers on the Harvard John A. Paulson School of Engineering and Applied Sciences (SEAS) have created a tentacle-like gripper that may grasp irregularly formed or tender objects with out damaging them.
The gripper is made up of many skinny, tender tentacles that depend on inflation to wrap themselves round an object with none sensing, planning or suggestions management. Individually, every tentacle is just too weak to choose up many objects, however with many working collectively the gripper can gently raise heavy and oddly formed objects.
Each tentacle is made up of a foot-long hole, rubber rubes. The tubes are made with thicker plastic on one facet, in order that was the tube is pressurized it curls like a pigtail. As the tube curls, it wraps and entangles itself round an object. Each added tentacle will increase the power of this maintain. The gripper releases the thing by merely depressurizing the tentacles.
When designing the gripper, the analysis staff took inspiration from nature. The gripper’s tentacles act equally to how a jellyfish stuns its prey.
To check how efficient the gripper was, the analysis staff used simulation and experiments the place the gripper was tasked with dealing with a variety of objects, together with totally different houseplants and toys.
The staff hopes that the gripper can be utilized to understand fragile objects, like tender vegatables and fruits in agricultural manufacturing and distribution and delicate tissue in medical settings, in addition to irregularly formed objects, like glassware, in warehouses. The gripper may exchange conventional grippers that depend on embedded sensors, advanced suggestions loops and superior machine-learning algorithms to work.
The staff’s analysis was printed within the Proceedings of the National Academy of Sciences (PNAS). It was co-authored by Clark Teeple, Nicholas Charles, Yeonsu Jung, Daniel Baum and James C. Weaver, and supported by the Office of Naval Research, the National Science Foundation, the Simons Foundation and the Henri Seydoux fund.