In a current examine posted to the Research Square* preprint server, researchers used a machine-learning (ML)-based mannequin to determine non-human CoVs (coronaviruses) which may trigger human infections.
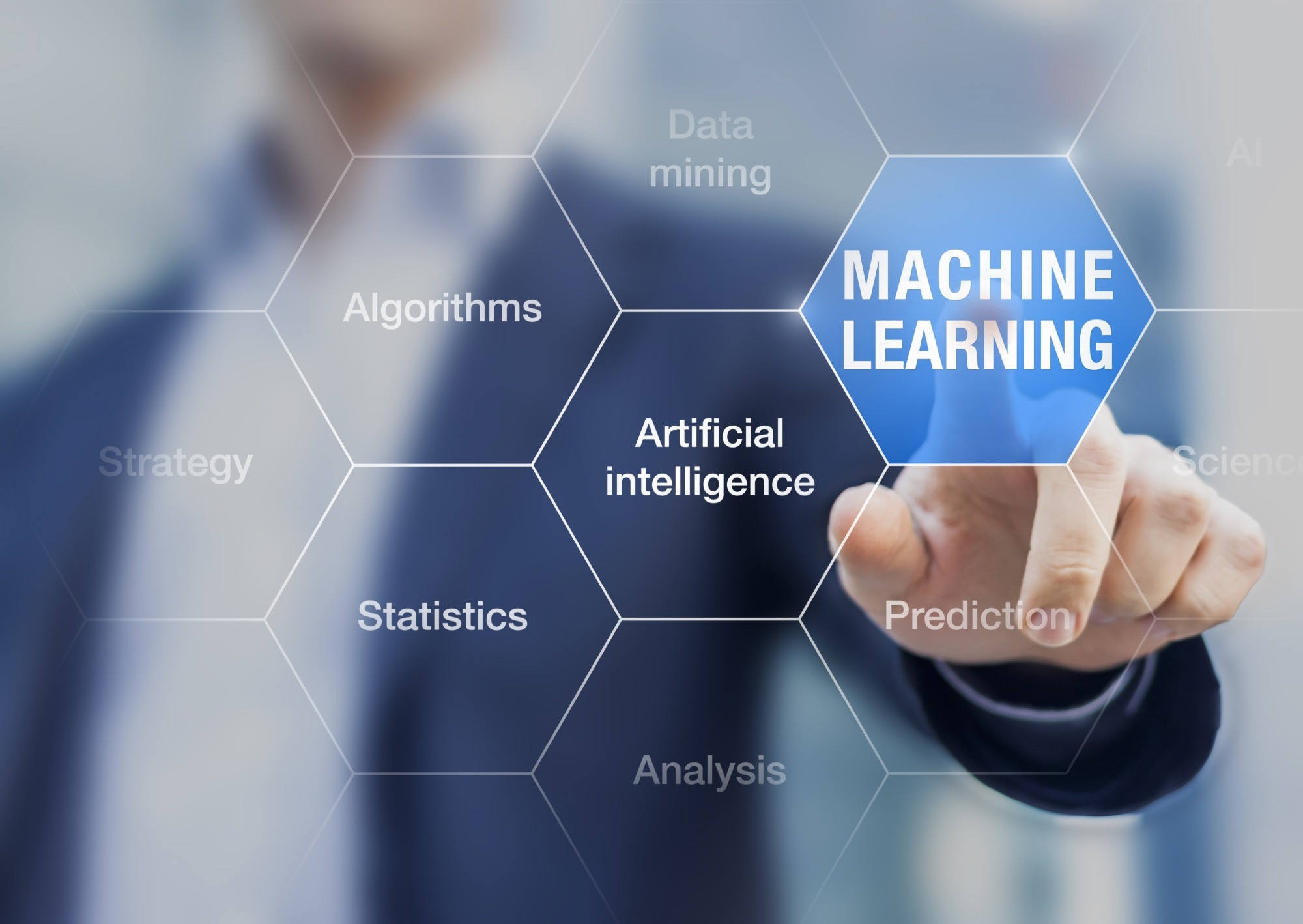
Background
Experimental knowledge is taken into account perfect for figuring out the host infectivity of a virus; nevertheless, your complete host vary for viruses is unknown. Several in-silico strategies have been used to estimate viral hosts, one primarily based on ML. Alignment-free strategies are reportedly preferable for in depth datasets comprising recombined virus sequences; nevertheless, such strategies don’t contemplate the relative location of contact residues within the sequences.
Studies have reported on shared alerts amongst totally different viral households for host estimation; nevertheless, restricted viral taxa have been included, not contemplating the distinctive virological traits and, due to this fact, stopping mechanistic research from being carried out on the pathways of host vary growth.
About the examine
In the current examine, researchers studied the infectivity of α-CoVs and β-CoVs in people by estimating binding interactions between the CoV S (spike) protein and human (host) receptors.
An ML-based mannequin was constructed with extreme acute respiratory syndrome coronavirus 2 (SARS-CoV-2) S protein sequences to estimate viral binding with host receptors. Skip-gram modeling was carried out utilizing synthetic neural networks to transform knowledge into vector format, such that the vectors code associations between the adjoining protein sequences. Further, the vectors are transformed, utilizing a logistic regression classifier, to human-binding potential (h-BiP) scores for the binding interactions between the protein sequences and host receptors.
The mannequin included 2,534 distinct α-CoV and β-CoV S sequences. Phylogenetic and MSA (a number of sequence alignment analyses) had been carried out. Molecular dynamic (MD) simulations of the S RBD (receptor-binding area) had been ready to judge virus-host receptor binding. The mannequin was retrained to research its software in host vary growth surveillance for viruses that emerged earlier than SARS-CoV-2. Pre-coronavirus illness 2019 (COVID-19) situations had been emulated by the exclusion of extreme acute respiratory syndrome coronavirus 2 sequences within the re-trained mannequin dataset.
The novel (re-trained) dataset comprised 1,369 CoVs, of which 540 confirmed human receptor binding. The coaching and testing datasets comprised human CoV (hCoV)-OC43, hCoV-NL63, hCoV-HKU1, the Middle East respiratory syndrome CoV (MERS-CoV), SARS-CoV-1, hCoV-229E, different MERS-associated viruses, different sarbecoviruses, different α-CoVs, different β-CoVs, and the porcine epidemic diarrhea virus. Viruses with h-BiP scores of ≥0.5 had been categorized as more likely to present human receptor binding.
Results
The ML mannequin produced h-BiP scores, primarily based on the S protein-host receptor binding of viruses that exactly evaluated the binding potential of human CoVs. The staff recognized two viral organisms, Bat CoV BtCoV/133/2005 (MERS-associated viral organism) and Rhinolophus affinis CoV isolate LYRa3 (SARS-associated viral organism) confirmed elevated h-BiP scores and beforehand unknown host receptor binding traits.
The findings indicated that the Bt133 virus and the LyRa3 virus had been related to non-human viral organisms with identified host receptor binding. The excessive sequence identification (97.0%) noticed for sharing of Bt133 S with Ty-HKU4 S indicated that the Bt133 virus binds with the human dipeptidyl peptidase 4 (hDPP4) receptor. Likewise, the 99% spike protein sequence identification between the LYRa3 virus and the LYRa11 virus indicated that the LYRa3 virus binds with the human angiotensin-converting enzyme 2 (hACE2) receptor.
MSA evaluation of RBMs (receptor-binding motifs) of the Bt133 virus and the LYRa3 virus with related viral organisms indicated that LYRa3 and Bt133 preserve their residues that contact host receptors. MD simulations validated the findings and recognized the residues in touch with the host receptors. Binding interactions had been noticed between the E518 residue in S RBD and the Q344 residue within the hDDP4 receptor and between the N514 residue in S RBD and the R317 residue within the hDDP4 receptor for Bt133. In addition, the Q515 contact residue was detected in >70.0% of Bt133 simulations. Contact residues for LYRa3 included T490, G492, Y485, and G486, detected in ≥45.0% of MD simulations.
In whole, 16 viruses with no identified host binding confirmed h-BiP scores of ≥ 0.5, indicating that the viruses would possibly bind to receptors on human cells. Of these, 14 viruses had been related to MERS and originated from dromedary camels of Africa. Previous research have indicated that MERS-CoV-associated viruses from camels in Africa would possibly trigger human infections.
The mannequin classifier confirmed 99.5%, 99.6%, and 98% accuracy, sensitivity, and specificity, respectively, and the h-BiP scores correlated nicely with the sequence identification findings. The h-BiP scores additionally discriminated between viral organisms with equivalent sequence identities, and the mannequin detected host receptor binding for low % sequence identification instances. Re-training of the mannequin additionally yielded related accuracy.
Overall, the examine findings confirmed the h-BiP-score-based ML method as an correct technique of estimating the human receptor-binding functionality of CoVs, underscoring that ML fashions could possibly be used to foretell host growth occasions.
*Important discover
Research Square publishes preliminary scientific stories that aren’t peer-reviewed and, due to this fact, shouldn’t be thought to be conclusive, information scientific apply/health-related habits, or handled as established data.