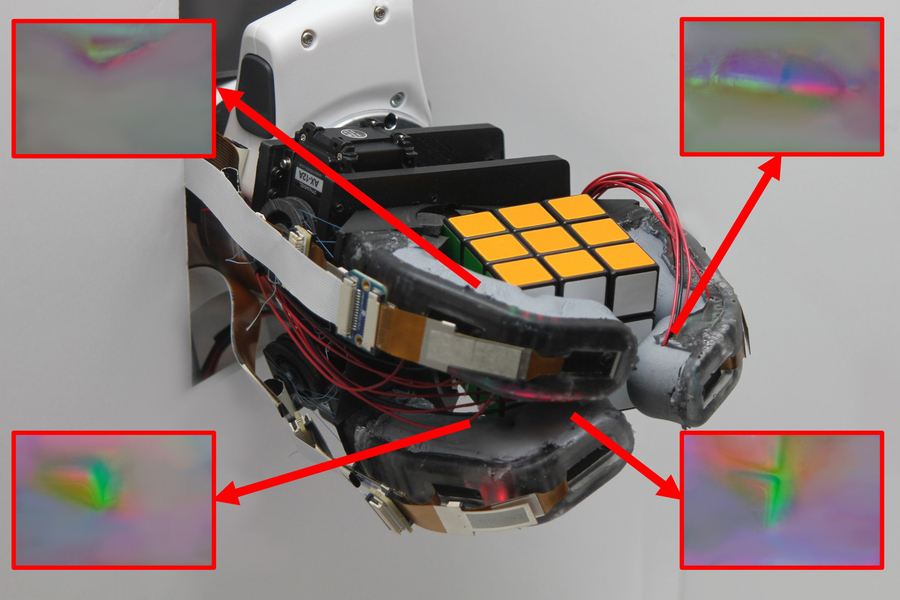
MIT researchers developed a soft-rigid robotic finger that includes highly effective sensors alongside its whole size, enabling them to supply a robotic hand that might precisely determine objects after just one grasp. Image: Courtesy of the researchers
By Adam Zewe | MIT News Office
Inspired by the human finger, MIT researchers have developed a robotic hand that makes use of high-resolution contact sensing to precisely determine an object after greedy it only one time.
Many robotic fingers pack all their highly effective sensors into the fingertips, so an object have to be in full contact with these fingertips to be recognized, which may take a number of grasps. Other designs use lower-resolution sensors unfold alongside all the finger, however these don’t seize as a lot element, so a number of regrasps are sometimes required.
Instead, the MIT workforce constructed a robotic finger with a inflexible skeleton encased in a delicate outer layer that has a number of high-resolution sensors integrated underneath its clear “skin.” The sensors, which use a digicam and LEDs to assemble visible details about an object’s form, present steady sensing alongside the finger’s whole size. Each finger captures wealthy knowledge on many components of an object concurrently.
Using this design, the researchers constructed a three-fingered robotic hand that might determine objects after just one grasp, with about 85 % accuracy. The inflexible skeleton makes the fingers sturdy sufficient to choose up a heavy merchandise, comparable to a drill, whereas the delicate pores and skin permits them to securely grasp a pliable merchandise, like an empty plastic water bottle, with out crushing it.
These soft-rigid fingers could possibly be particularly helpful in an at-home-care robotic designed to work together with an aged particular person. The robotic may carry a heavy merchandise off a shelf with the identical hand it makes use of to assist the person take a shower.
“Having both soft and rigid elements is very important in any hand, but so is being able to perform great sensing over a really large area, especially if we want to consider doing very complicated manipulation tasks like what our own hands can do. Our goal with this work was to combine all the things that make our human hands so good into a robotic finger that can do tasks other robotic fingers can’t currently do,” says mechanical engineering graduate scholar Sandra Liu, co-lead creator of a analysis paper on the robotic finger.
Liu wrote the paper with co-lead creator and mechanical engineering undergraduate scholar Leonardo Zamora Yañez and her advisor, Edward Adelson, the John and Dorothy Wilson Professor of Vision Science within the Department of Brain and Cognitive Sciences and a member of the Computer Science and Artificial Intelligence Laboratory (CSAIL). The analysis might be introduced on the RoboSoft Conference.
A human-inspired finger
The robotic finger is comprised of a inflexible, 3D-printed endoskeleton that’s positioned in a mildew and encased in a clear silicone “skin.” Making the finger in a mildew removes the necessity for fasteners or adhesives to carry the silicone in place.
The researchers designed the mildew with a curved form so the robotic fingers are barely curved when at relaxation, identical to human fingers.
“Silicone will wrinkle when it bends, so we thought that if we have the finger molded in this curved position, when you curve it more to grasp an object, you won’t induce as many wrinkles. Wrinkles are good in some ways — they can help the finger slide along surfaces very smoothly and easily — but we didn’t want wrinkles that we couldn’t control,” Liu says.
The endoskeleton of every finger incorporates a pair of detailed contact sensors, often called GelSight sensors, embedded into the highest and center sections, beneath the clear pores and skin. The sensors are positioned so the vary of the cameras overlaps barely, giving the finger steady sensing alongside its whole size.
The GelSight sensor, based mostly on expertise pioneered within the Adelson group, consists of a digicam and three coloured LEDs. When the finger grasps an object, the digicam captures photographs as the coloured LEDs illuminate the pores and skin from the within.
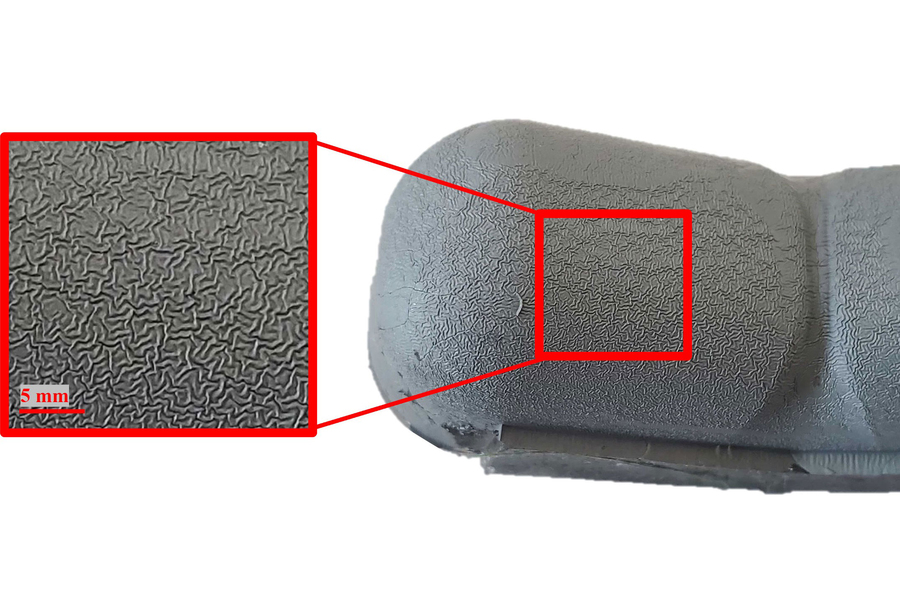
Image: Courtesy of the researchers
Using the illuminated contours that seem within the delicate pores and skin, an algorithm performs backward calculations to map the contours on the grasped object’s floor. The researchers educated a machine-learning mannequin to determine objects utilizing uncooked digicam picture knowledge.
As they fine-tuned the finger fabrication course of, the researchers bumped into a number of obstacles.
First, silicone tends to peel off surfaces over time. Liu and her collaborators discovered they may restrict this peeling by including small curves alongside the hinges between the joints within the endoskeleton.
When the finger bends, the bending of the silicone is distributed alongside the tiny curves, which reduces stress and prevents peeling. They additionally added creases to the joints so the silicone shouldn’t be squashed as a lot when the finger bends.
While troubleshooting their design, the researchers realized wrinkles within the silicone forestall the pores and skin from ripping.
“The usefulness of the wrinkles was an accidental discovery on our part. When we synthesized them on the surface, we found that they actually made the finger more durable than we expected,” she says.
Getting grasp
Once they’d perfected the design, the researchers constructed a robotic hand utilizing two fingers organized in a Y sample with a 3rd finger as an opposing thumb. The hand captures six photographs when it grasps an object (two from every finger) and sends these photographs to a machine-learning algorithm which makes use of them as inputs to determine the article.
Because the hand has tactile sensing overlaying all of its fingers, it could collect wealthy tactile knowledge from a single grasp.
“Although we have a lot of sensing in the fingers, maybe adding a palm with sensing would help it make tactile distinctions even better,” Liu says.
In the long run, the researchers additionally wish to enhance the {hardware} to scale back the quantity of damage and tear within the silicone over time and add extra actuation to the thumb so it could carry out a greater diversity of duties.
This work was supported, partly, by the Toyota Research Institute, the Office of Naval Research, and the SINTEF BIFROST undertaking.
MIT News