Enterprises see essentially the most success when AI tasks contain cross-functional groups. For true affect, AI tasks ought to contain information scientists, plus line of enterprise house owners and IT groups.
By 2025, based on Gartner, chief information officers (CDOs) who set up worth stream-based collaboration will considerably outperform their friends in driving cross-functional collaboration and worth creation.1 In order to drive this type of AI success, you want a cross-functional group engaged within the course of, invested in outcomes, and feeling a way of duty alongside the whole lifecycle.
You can construct your AI group with individuals from throughout your group, together with:
- AI leaders who’re answerable for AL/ML technique and the roadmap inside a corporation;
- AI builders who’re answerable for AI technique implementation and search to handle enterprise issues utilizing machine studying;
- Business executives who look to unravel enterprise issues and drive income or scale back prices with AI;
- and IT leaders who’re centered on the expertise infrastructure of a corporation, together with the information and analytics infrastructure.
Quite just a few advanced use instances, equivalent to worth forecasting, would possibly require mixing tabular information, photos, location information, and unstructured textual content. When you have got messy information coming from in every single place, you want a robust AI platform as a way to transfer ahead and implement your AI.
In addition, it’s important that fashions adjust to laws and deal with prospects pretty, making it extra essential than ever to watch fashions in manufacturing. It is feasible to handle the end-to-end AI lifecycle in a single resolution. The DataRobotic AI Platform makes it potential to have interaction your cross-functional group to ship profitable AI outcomes, irrespective of how advanced your inputs.
The price of actual property has been a rollercoaster journey on this difficult macroeconomic local weather. In this instance, we take a deep dive into how actual property corporations can successfully use AI to automate their funding methods.
We additionally have a look at how collaboration is constructed into the core of the DataRobotic AI platform in order that your whole group can collaborate from enterprise use case to mannequin deployment. Let’s check out an instance use case, which showcases the efficient use of AI to automate strategic choices and explores the collaboration capabilities enabled by the DataRobotic AI platform.
Improving Productivity with Increased Collaboration
We begin by exploring a dataset from the DataRobotic AI catalog. The DataRobotic AI catalog fosters collaboration by offering customers a system of report for datasets, the power to publish and share datasets with colleagues, tag datasets, and handle the lineage of the dataset all through the whole mission. In essence, the AI catalog permits you to crowdsource datasets in a means that’s extremely related to your enterprise, utilizing already present property to construct fashions which can be most helpful to your enterprise.
AI catalog encourages a tradition of collaboration and sharing information property that may profit your group, resulting in large good points in productiveness, sharing new sources, and making a collaborative setting for enterprise AI.
You can even handle entry management and sharing permissions to those datasets, in case you’re coping with delicate information that needs to be accessible solely to a restricted variety of stakeholders.
Estimating Asset Value Using the DataRobotic AI Platform
According to the Federal Housing Finance Agency, the U.S. worth index rose by 19.17% yr over yr in 2021, which was a big enhance from the prior yr’s 6.92% development—so massive that it was the best annual development on report.
In such a scorching market, how can groups leverage AI to make sure that they’re assessing the appropriate values of their respective markets? The demo from the session highlights distinctive and differentiated capabilities that empower all customers—from the analysts to the information scientists and even the individual on the finish of the journey who simply must entry an immediate worth estimate.
In our demonstration, we utilized an actual property dataset from Ontario which included previous gross sales information of properties. Our goal was to create a machine studying mannequin that would precisely predict the promoting worth of a single-family dwelling.
When contemplating a property, we take note of a number of elements equivalent to its location, dimension (sq. footage), and the variety of bedrooms and loos. Additionally, we additionally analyze unstructured info equivalent to what facilities include the property, for instance a sauna or lighting fixtures, and assessment accompanying pictures. By analyzing all of this info, we intention to achieve insights and decide an estimated promoting worth for a brand new property.
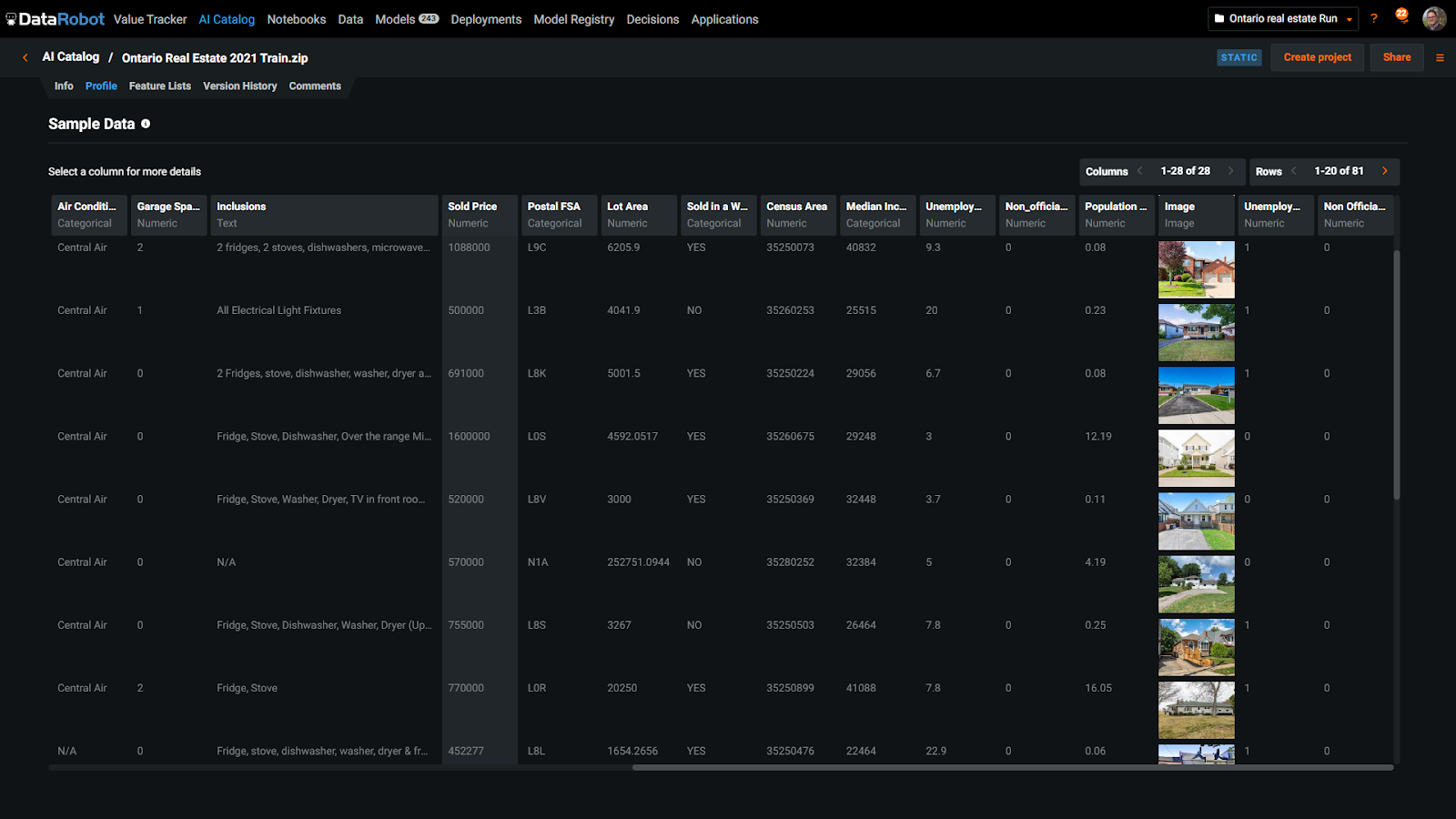
The actual property market modifications over time, so it’s essential that our mannequin learns from previous information and is examined on a timeframe from the longer term. DataRobotic helps you automate this backtesting by establishing Out-of-Time Validation that forces your mannequin to study from information earlier than a sure date after which validate towards information that comes after that cut-off level.
When working with location-oriented information like homes in a neighborhood, a functionality that actually helps inside DataRobotic is Automated Geospatial Feature Engineering that converts latitude and longitude into factors on the map. These factors drive a characteristic engineering course of that clusters close by houses collectively and calculates many values equivalent to the common promoting worth in that location.
Automated Feature Discovery is one other differentiator that may have an effect on this use case. It permits us to simply mix information from different sources and summarize it on the unit of research of our mission. In this instance, now we have census information on the neighborhood and metropolis stage which DataRobotic will incorporate into our mission on the property stage. Also, it should routinely compute transferring aggregations, equivalent to the common worth by neighborhood for the final week, month, and three months. These information preparation duties are in any other case time consuming, so having DataRobotic’s automation right here is a large time saver.
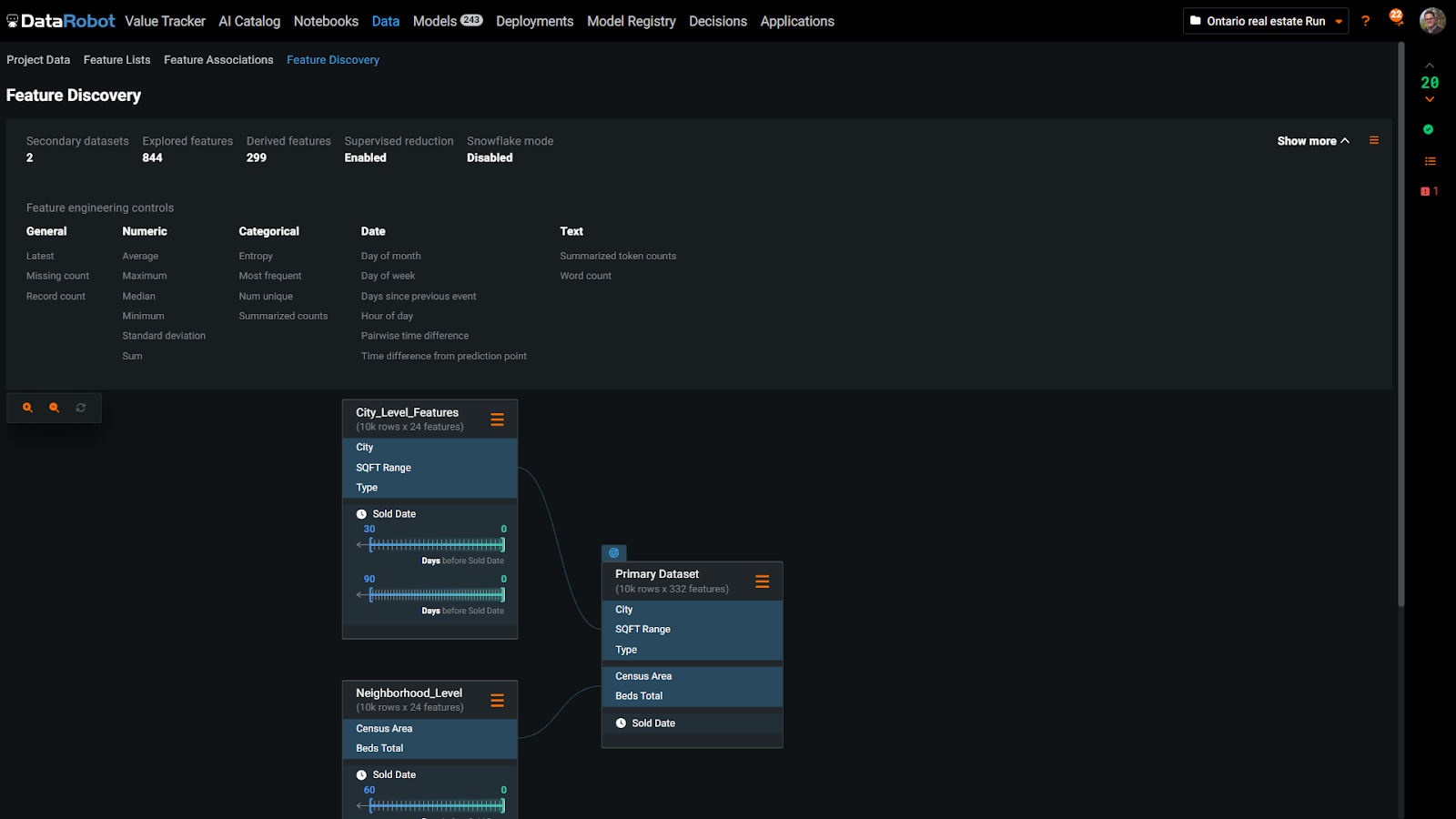
After establishing your mission, you may get began. Hit the Start button, and DataRobotic will start exploring huge combos of characteristic engineering steps and machine studying fashions. Automated characteristic engineering reveals many insights by creating new options from present ones. This helps with getting extra inventive along with your experimentation.
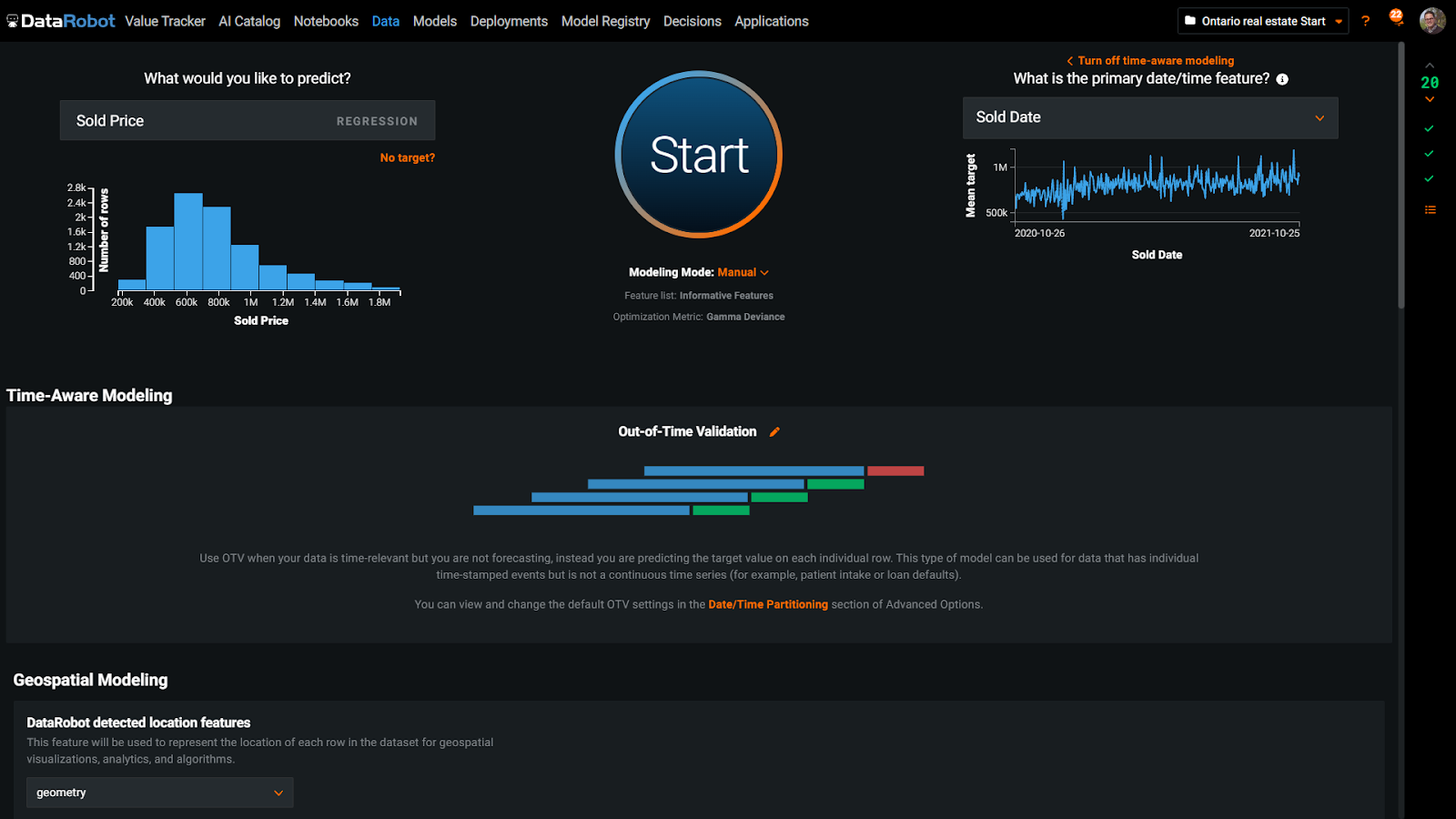
As we run the mannequin, we see that taking the 90-day median of the offered worth on the metropolis stage was a helpful predictor. DataRobotic does a fantastic job of explaining precisely the way it bought to this characteristic. It joins the first information with the city-level dataset and calculates the transferring 90-day median.
Delivering Explainable and Transparent Models with DataRobotic
Explainability is a key differentiator in DataRobotic that enables for smoother collaboration in your group. DataRobotic additionally supplies a number of instruments for understanding the habits of the mannequin and gaining perception into why predictions are generated as they’re. Feature Lineage, Feature Effects, Prediction Explanations, and SHAP (SHapley Additive exPlanations) enable for a complete examination of the mannequin’s underlying logic and decision-making processes. These instruments present beneficial info on the relationships between options and predictions, enabling information scientists to make knowledgeable choices when fine-tuning and bettering their fashions.
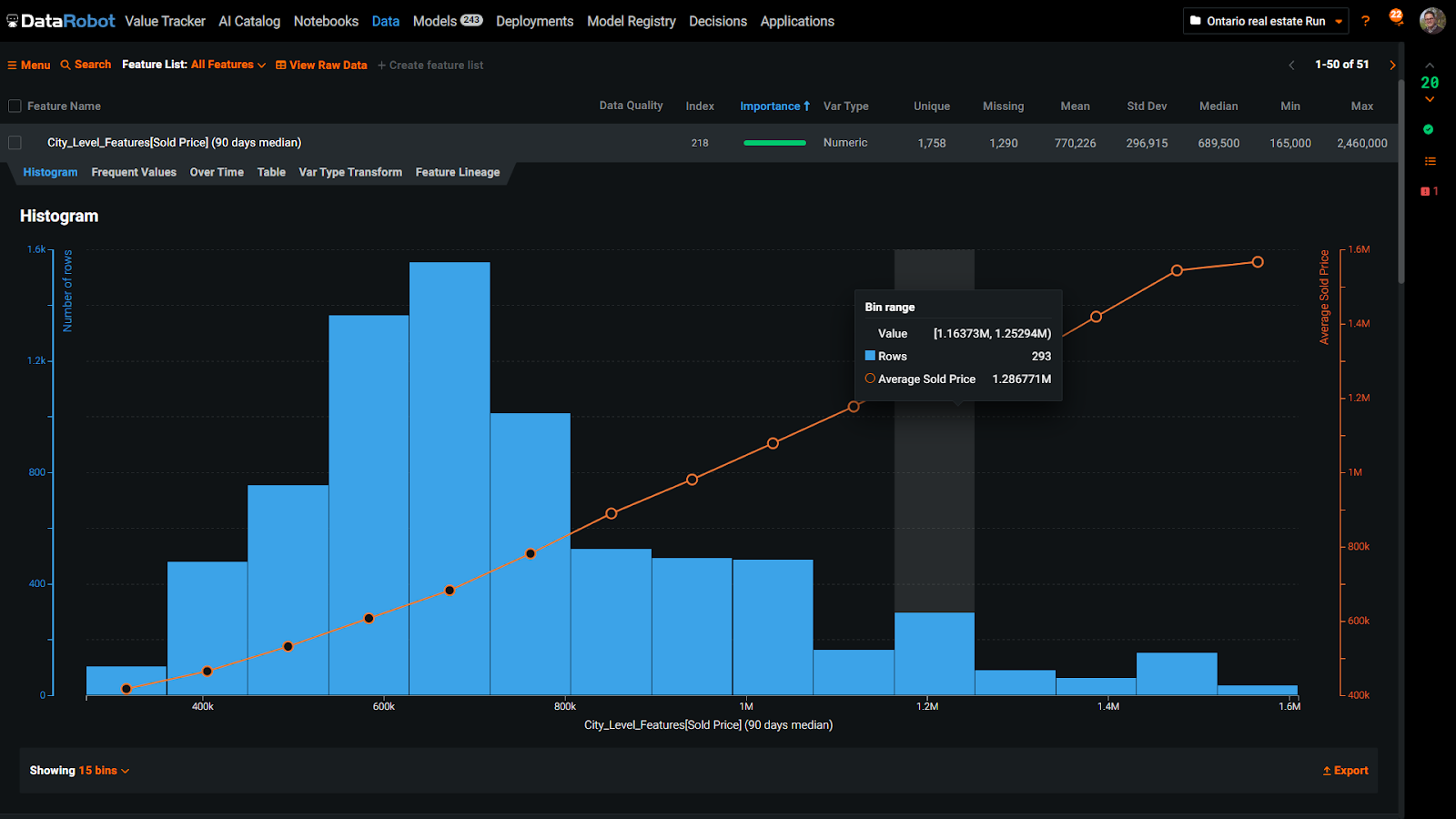
DataRobotic supplies a leaderboard displaying outcomes from totally different experiments, together with a various vary of algorithms, preprocessing, and have engineering. The algorithm blueprint, together with all steps taken, might be considered for every merchandise on the leaderboard. This permits information scientists to simply evaluate approaches and select the most effective mannequin for his or her wants.
In every blueprint, customers could make customized modifications through drag and drop or code, to check their very own concepts, aided by DataRobotic’s security guardrails. As experiments progress, DataRobotic supplies insights by way of its use of location options. It highlights the areas the place predictions had been correct and people the place the mannequin struggled. This info helps information scientists perceive the place enhancements might be made by figuring out errors and incorporating extra information.
After coaching a mannequin, it is very important assess its equity. DataRobotic provides the power to judge bias by conducting a bias and equity evaluation. By incorporating census information, equivalent to language or unemployment info, DataRobotic can decide if sure neighborhoods are unfairly handled in comparison with others. The evaluation might uncover attributes that enhance accuracy however negatively affect equity. To tackle this concern, DataRobotic supplies the power to handle bias by putting higher emphasis on underrepresented options, bettering equity and enhancing the trustworthiness of the AI mannequin.
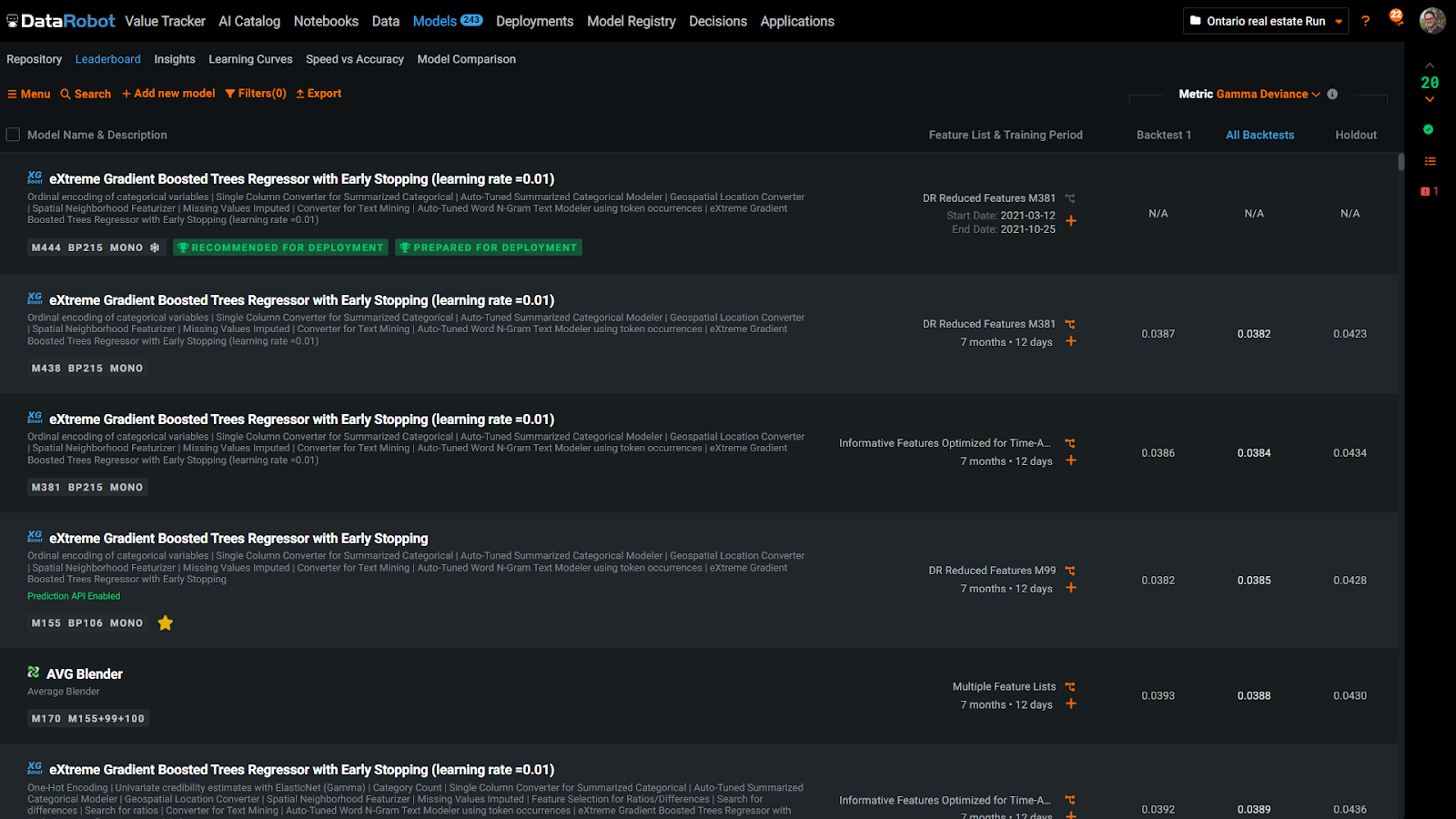
DataRobotic makes it easy to take your mannequin reside. With only one click on, your mannequin might be containerized and accessible by way of an API endpoint. The MLOps command middle provides you a birds-eye view of your mannequin, monitoring key metrics like accuracy and information drift. The Accuracy tab particularly reveals how the mannequin’s accuracy has modified since deployment, serving to you retain monitor of its efficiency within the real-world.
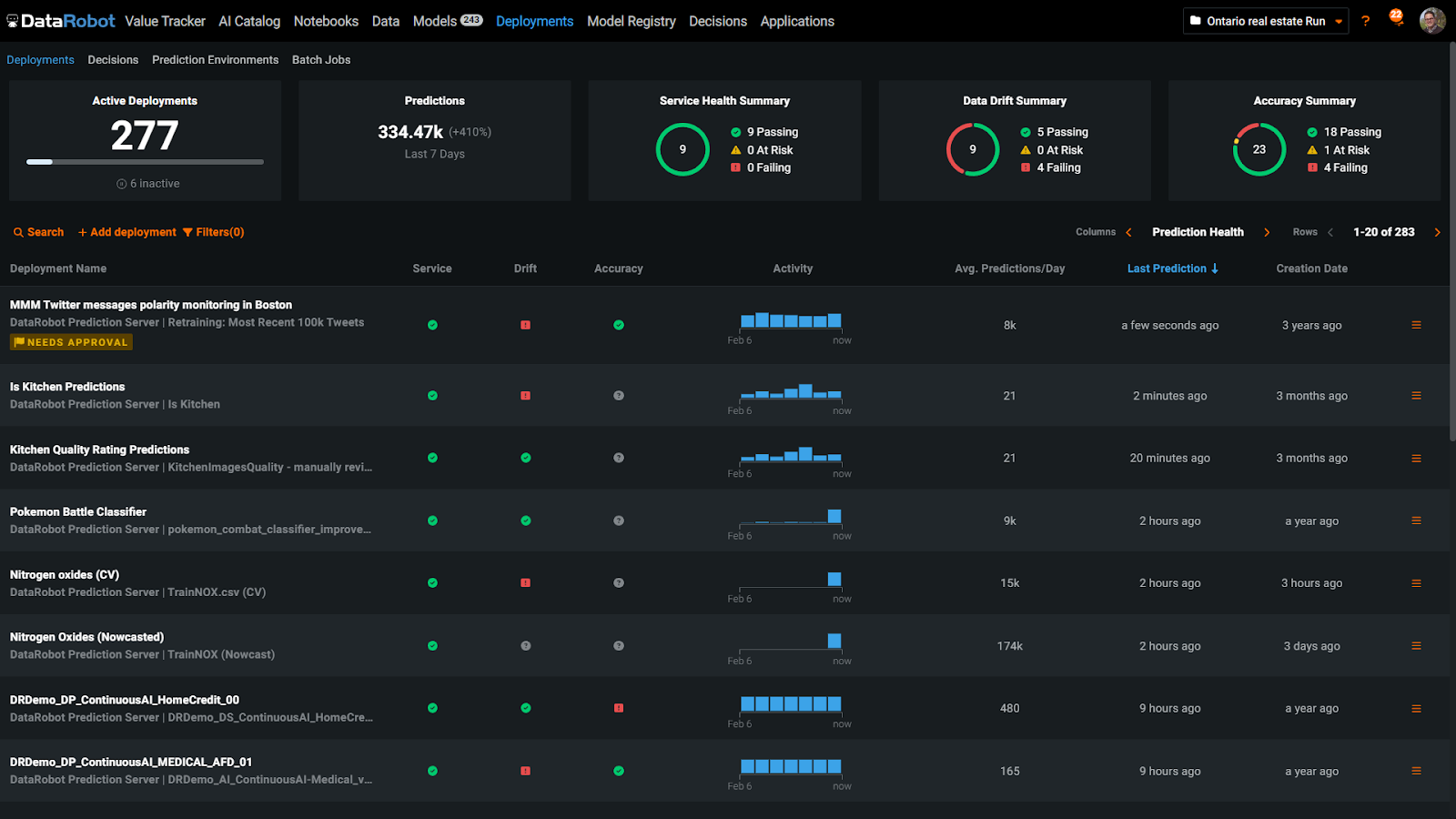
The Data Drift tab shows a scatter plot of the mannequin’s enter options, providing a real-time glimpse into the information the mannequin is utilizing to make predictions, equivalent to the kind of flooring, proximity to varsities, or the outside of the house. This illustration demonstrates that the mannequin is encountering dwelling exterior varieties that weren’t a part of its coaching information, which may result in surprising outcomes and decreased accuracy. To stop this, alerts like this function a reminder to retrain the mannequin, an motion that may simply be automated inside DataRobotic.
After retraining the mannequin, DataRobotic will change the outdated mannequin with the up to date model. Additionally, you possibly can add the newly retrained mannequin as a challenger, permitting you to match the efficiency of each fashions throughout numerous metrics. The choice to designate the brand new mannequin because the champion can also be out there, enabling you to judge their relative strengths and weaknesses.
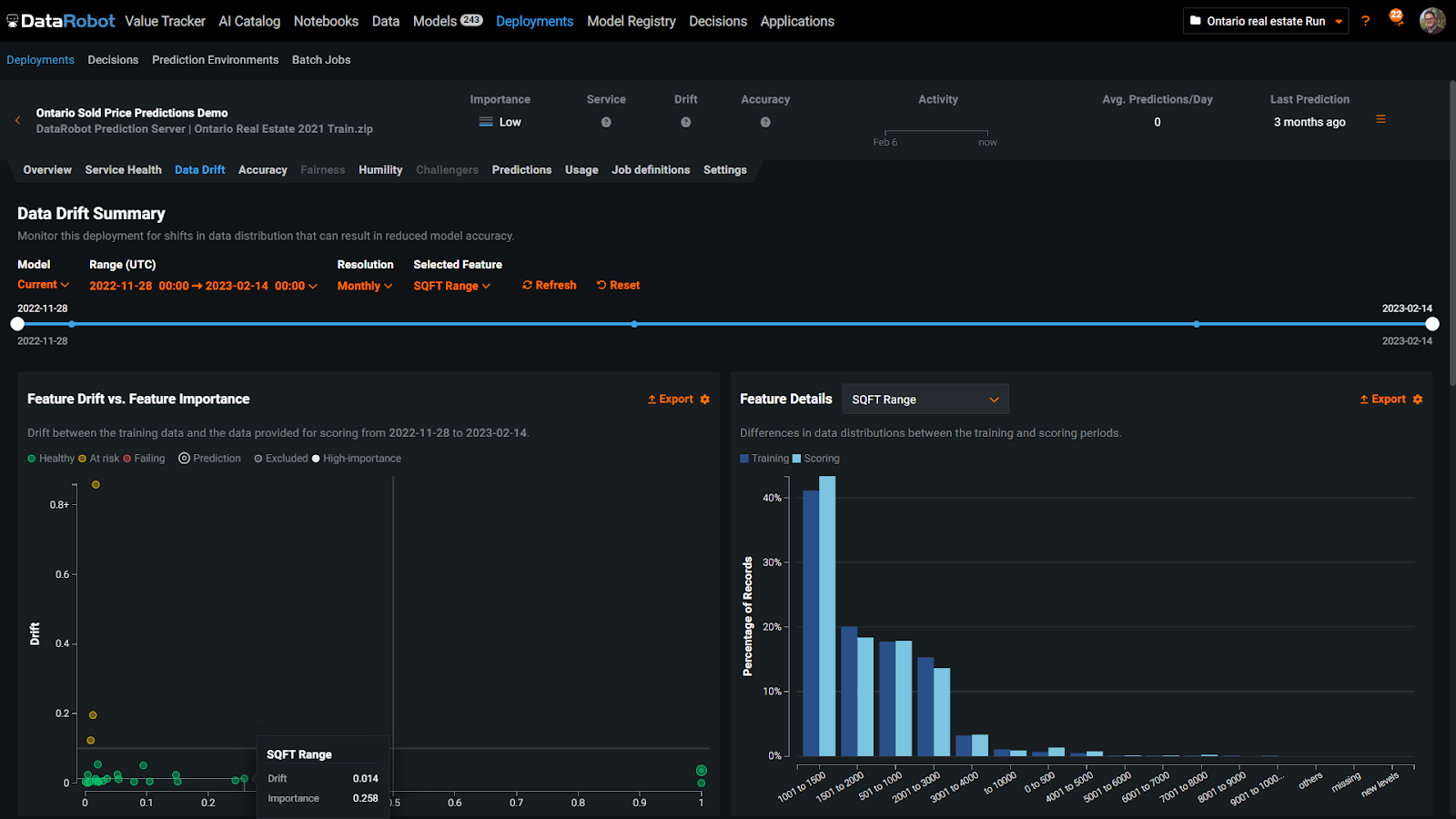
Finally, you possibly can generate an software that serves because the front-end for the mannequin, permitting customers to enter variables and get predictions. These enterprise purposes might be shared with anybody, enhancing their capacity to make knowledgeable real-world choices.
DataRobotic Gives Your Team End-to-End Automation, Accuracy, and Fairness
The DataRobotic AI Platform empowers your group with options and capabilities that remedy among the most urgent issues groups face when implementing AI. The platform permits your group to wash up information, make changes, run experiments, acquire insights, guarantee equity, and deploy the mannequin to finish customers— optionally with out writing a line of code. DataRobotic can even join various kinds of information, together with geographic and time sequence information.
With DataRobotic Automated Feature Engineering, your group can streamline the method of mixing exterior datasets and save time by consolidating and getting ready information for mannequin constructing. This characteristic helps simplify the mannequin constructing course of, getting higher outcomes sooner.
With DataRobotic MLOps, you possibly can deploy, monitor, and handle your manufacturing mannequin with ease. Teams can even construct AI apps with out writing code and collaborate inside a single system of report, establishing person permissions and governance. This simplifies the AI growth course of, liberating up information scientists to concentrate on extra strategic duties.
Leading enterprises worldwide depend on DataRobotic to ship profitable AI tasks, managed by cross-functional groups together with information scientists, IT infrastructure specialists, and enterprise items. Effective teamwork and clear communication are key to making sure a easy, seamless, and profitable course of.
1Gartner, How to Overcome the Top 6 Roadblocks to D&A Leader Success, Jorgen Heizenberg, Carlie Idoine, May 4 2022
About the writer
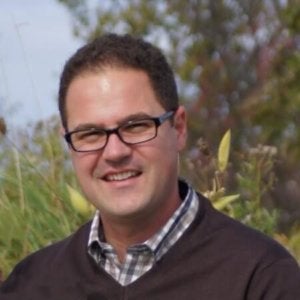
Senior Data Scientist, Team Lead at DataRobotic
Brett Olmstead is a Sr. Data Scientist at DataRobotic main the Customer Facing Data Science group in Canada. Brett has 15 years of expertise implementing predictive analytics options with shoppers throughout many industries. Prior to DataRobotic he helped launch the startup DataScience.com, which was acquired by Oracle in 2018. At DataRobotic, he’s busy serving to prospects change into wildly profitable with their Machine Learning and AI tasks utilizing the DataRobotic Platform.